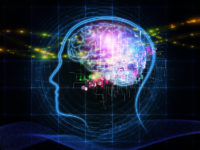
Using machine learning to improve your product

This post was written by Rose Yao, and was first published on Code Like A Girl.
One of my side projects this year has been to get comfortable with Machine Learning. I took Andrew Ng’s course, spent some time playing with tensorflow and prototyped some ideas around object recognition. I was curious to get in deep enough technically to understand the limitations and the challenges of the tech stack right now. Everywhere we look, there’s a startup promising to use machine learning to do wondrous things. From autonomous cars to the smarter medical diagnosis to perfect live translations. Yet unlike mature platforms like iOS, Android, and the Web, it’s very unclear how to get started and what the building blocks are.
My goal is to understand if you do not have the teams of phDs or massive datasets like Google, Facebook, Amazon, or Apple, how do you use machine learning to improve a product or idea? What are the opportunities right now?
The Basics
- This is a big deal now because while the algorithms behind machine learning hasn’t changed in many years, the amount of data and computing power available has increased significantly. Making things that seems impossible 10 years ago accessible on the average smartphone.
- Machine learning is 90% math. The simplest version is just fitting data to a curve. Example give a machine learning engine a set of data that includes: square footage, and housing price. It will try to find a curve that can be used to predict housing prices based on the square footage of the house. Most problems are the more complex multi variable version of that.
- Deep learning means tiers of machine learning networks that work together. Similar to logic gates, simple and/or gates can only do so much, but linked together they’re almost limitless. Traditionally a ml network just had an input and and output layer. Deep learning just means adding hidden layers to help refine the final output.
- Be comfortable with the black box: One of the big breakthroughs is when we stopped trying to get computers to think like we do. For example, we used to try to teach the computer to see the way we do. Step 1 understand basics (shapes, colors, texture), then what shapes make an object (plants, animals, people), then maybe in plants what flowers are vs trees vs grass. This was frustrating for everyone involved. Modern machine learning just gives the network inputs and outputs. The humans are in charge of picking the right inputs, making sure the data is well labeled, what type of network or algorithm is best for the problem, how complex the network is and how to best train it. The rest is one giant black box. Debugging is very different, there’s no tracing where things went wrong like a normal codebase.
How do you get started?
Step 1: Decide what problem are you interested in solving?
As always in product strategy, start with what the problem is then decide the best technology to solve that problem. It may or may not involve machine learning. Here’s a subset of common problems that machine learning is great for.
Computer Vision: Any problem that involves analyzing or manipulating an image. Examples: Identify all the people in a photo and automatically know their gender and age. Identify a glass of wine and overlay information about what it pairs well with.
- Difficulty: Low. There are lots of reasonably inexpensive server side solutions . There’s also a ton of excitement around this area, probably because the results are so tangible. To train a custom network, lots of people have experimented with inception and in my own experience if you’re not afraid of Terminal, Python, and Stack Overflow, you’ll be able to build a custom network in a week or less.
- Data availability: High. A custom category on an existing network like inception needs ~100 images. This is a pretty low bar to entry given how many well labeled public photos are floating around the internet .
Text: How do I understand a lot of text based information without human help? How do I go beyond key words and basics and really understand meaning/trends? I haven’t played with this a lot personally but luckily it is a common problem. Translation is a highly specialized subset in this area.
- Difficulty: Low. Similar to computer vision. There’s a lot of good APIs. However quality varies widely so it really depends on how specific your problem is.
- Data availability: Medium. Same as images, the Internet has made human created content widely available. The problem is that they’re not always well labeled. Manual labeling might be expensive and time consuming.
Speech: Using voice or sound as input or output in some way. The most obvious examples are assistants like Siri or the smart home systems like Alexa or Google Home.
- Difficulty: Medium. For basic things like a single player speaking clearly in a microphone, there are good APIs. But more complex problems like dealing with multiple speakers in a noisy environment are unsolved.
- Data availability: Low. Getting clean labeled data (the holy grail of machine learning) is very challenging. Sound files with words and people labeled precisely are in short supply. (Much harder than cat photos.)
Recommender systems: Almost every company I work with can benefit from a good personalized recommender system. This is the classic problem of based on what a customer buys/likes/views, they might like these other things. This applies to commerce, to content, and to social products.
- Difficulty: Medium. I’m shocked to say there’s not a large set of plug and play APIs for this. (opportunity?) Most likely it’s because datasets in this category are so diverse and the inputs are so specific. The best I’ve seen is AWS which has some basic abstractions and since most startups use AWS for data, it makes it much easier to pass in inputs.
- Data availability: Unique. Most likely this is proprietary data and there’s a lot of it.
Predictive systems: This problem involved given a bunch of data how likely is an outcome? For example, given a lot of historical data on baseball teams, who will win this season and by how much?
- Difficulty: Medium. Again, not a ton of plug and play solutions here. AWS has a solution here that doesn’t require creating a custom network using tensor or another toolkit but it still requires some understanding on how to setup and train a network.
- Data availability: Unique. Most likely this is proprietary data and there’s a lot of it. Be careful here with systems that are self influenced. For example predictive systems for the stock market don’t work well or they don’t work for long because as soon as the prediction becomes known by enough people, the market adjusts for that expectation.
Step 2: Decide if Machine Learning is just a tool or if it is the key differentiator for the company.
I listed a few common problems that machine learning can solve. It can take a cumbersome experience like maybe onboarding a new user and make it delightful and seamless. However, that in itself is NOT a key differentiator. It is just a tool. Machine learning can be a unique competitive advantage when there is:
- Unique data and industry knowledge: There are lots of companies that are sitting on incredibly valuable data and applying machine learning to that data set can change the business. For example: insurance, healthcare, ISPs, etc.
- Unique technical insight or talent: we’ve barely scratched the surface on the problems we can apply machine learning to. Whether it’s speech or sound or video analysis, the best machine learning networks are no where as smart or capable as a human being. There’s a lot of room to improve. For a team of engineers with experience with machine learning and unique technical insight, solving any facet of one of these big problems can be incredibly valuable.
Step 3: Build it!
I’m not the first one to say this, but I believe that in the next 5 years, basic machine learning techniques will be integrated into almost every product we use. Just like toddlers today assume every surface is “touchable”. We will start to have expectations around how smart our products are. So go build something, big or small. Think about how a little AI can improve a product. There are lots of toolkits, Kenneth does a great job of explaining the pros/cons of each toolkit. I personally prefer tensorflow because most error messages have stack overflow pages. 🙂
Happy Hacking!
As always contact me if you have feedback or need help!
Imagery by A Health Blog, made available under an Attribution 2.0 Generic license