In the Advanced stage of product analytics maturity, data is your compass for most decisions. This analysis is driving your product development because you’re constantly considering what works, for who, and why.
You’ve gotten in shape with your dumbbells and bench press and mile-long runs. Now it’s time to join a gym. Something that allows you to choose the equipment or exercise that will strengthen the muscle group of your choice and/or lead to greater endurance.
Most (or all) decisions are made with product analytics in mind, and are supported by real-time data. All teams have access to product data, and your Product, Engineering, and Design teams will rely on it heavily.
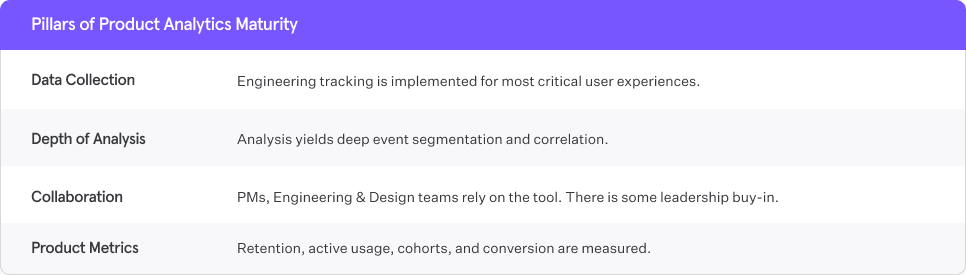
Common markers of Advanced stage
In this stage, businesses use multiple user journey solutions. These include attribution, engagement, and experimentation tools that are mostly integrated, requiring very few manual workflows.
The automation means you can more easily pull product analytics into your decision workflow. At the same time, because most user experiences are tracked, you have access to a much deeper dataset. Careful planning is critical at this stage in order to leverage the data instead of getting overwhelmed by it.
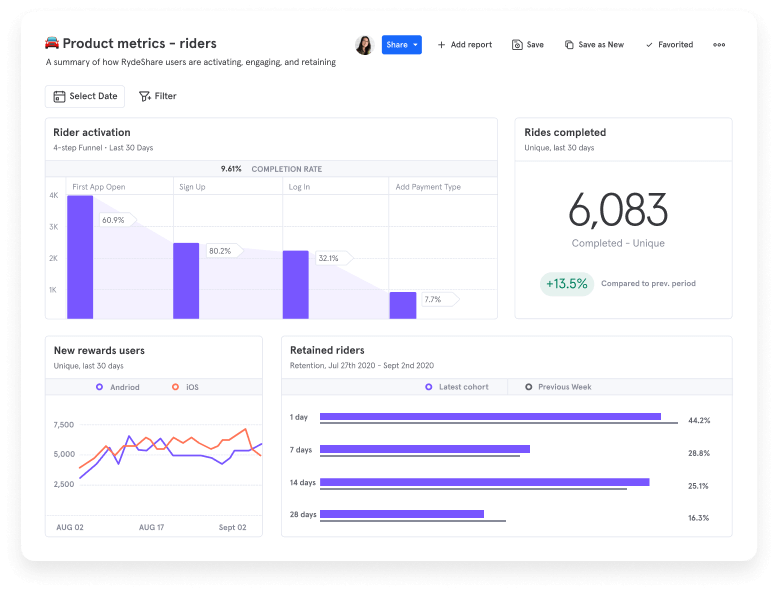
Explore Mixpanel
See how product and growth teams use Mixpanel’s powerful reports to answer any question.
Try It With Sample DataExample of Advanced
product analytics maturity
Questions you can answer
In the Advanced stage, you can assess what’s happening to your product and identify factors contributing to these patterns:
- What combination of user attributes (like device or region) lead to the highest product retention rates?
- Who are my power users and how does their usage differ from that of casual users?
- What sequences of actions lead my users to upgrade their membership?
- Which in-product actions correlate most strongly with high retention, a plan upgrade, or any other goal?
- How do engagement and retention vary by company or account?
In this stage, businesses not only have a solid grasp on how to collect and utilize data, but analytics is woven into the fabric of their culture. Employees are curious, ask questions, and are encouraged to use tools to make iterative improvements.
For ExampleEventually, the ridesharing app collects sophisticated data: type of ride requested (e.g., SUV vs. shared, length of ride, rider interactions vs. driver interactions) and finds a tool that supports advanced metrics and analysis.
This product analysis informs and guides future changes to the app. They regularly consult what data they have to make sure that what they’re doing lines up with what they should be doing.
They implement the right tech stack for improving their user experience and for making decisions based on product analytics.
Because they’re using data well, their development is more effective and they waste less time and money on features that might not be well received. There is still some manual upkeep (e.g., tracking new events as new features and functions are released) necessary to maintain their product analytics implementation, but it’s kept to a minimum and well worth the effort.
How to move on from Advanced
Advanced product analytics maturity means that your company knows the value of data and uses it to guide product decisions. However, there’s still a good chance that your analytics process has a few gaps that require a manual workflow.
This may seem like a good place for many companies to stop with their adoption of product analytics. But moving to Expert product analytics capabilities means that you can dig deep into the impact of individual marketing campaigns and feature updates on product and company KPIs.
To achieve the Expert stage, all parts of the process need to be automated by a quality tool. More importantly, the product analytics and metrics should be guiding all, not just most, product decisions. At the Expert level, companies begin to implement machine learning and data science.