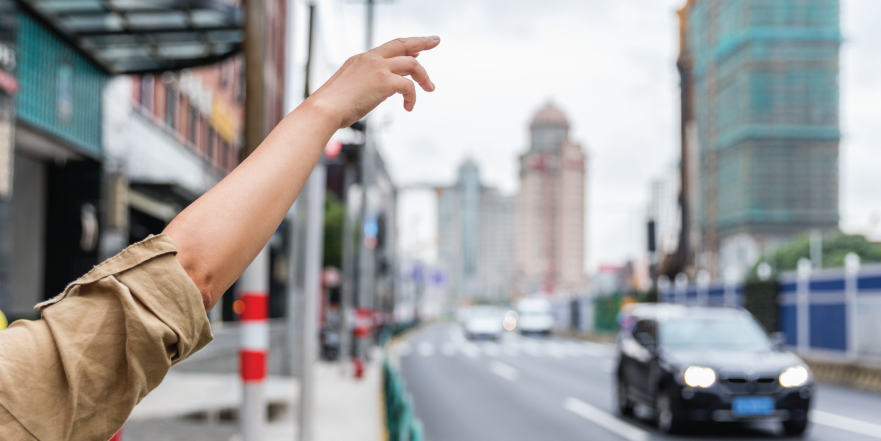
George Xing shares the data strategy behind Lyft’s explosive growth

When it comes to talking data strategy, George Xing doesn’t mince words: “Data analysts do more than just produce ‘interesting insights.’’’ Companies should be able to measure the performance of their data and analytics teams quantitatively. Like any other employee, analysts should be incentivized to make positive business impact.”
George has kept this philosophy at the fore as he leads the rapidly growing analytics team at Lyft, the ride-sharing service now completing over one million rides a day. In the last three years, George has scaled analytics from four people to forty-five and is pushing forward with a plan to double the team in size by the end of next year.
As George led us through the winding hallways of the company’s still-under-construction HQ, it became apparent that transformation is part and parcel of daily life at Lyft. In our interview, George explains how he set up Analytics to ride – and even drive – the sea-change. Along the way, he shares four tenets behind the data strategy that has made Lyft’s explosive growth possible.
Eliminate barriers to high-impact work
Analytics teams have more business problems than time or resources to solve them. For that reason, prioritization is critical.
To ensure that the most impactful business problems would get analyst attention, George built a decision framework – the kind whose answers can solve problems that touch almost every user at least once. “I often like to use a pyramid analogy with my team. There is a wide base of questions that are important for keeping the lights on but don’t necessarily improve how we run the business. There is a much smaller number of more challenging but highly impactful questions at the top. As a team, we try to focus on these first, while enabling other teams to answer as many of the others ones as possible.”
The questions at both the top and bottom of the pyramid change as businesses mature. In the early days of Lyft, when analytics resources were stretched thin, George focused the team on those few problems that would significantly move the needle and provide a scalable solution for other teams.
An early example of this involved working with a distributed set of local operations teams in charge of Lyft’s largest markets. “First, we mapped out the mechanics of the business in each market. Then we designed a decision-making framework that allowed us to provide growth and budget recommendations to each city’s general manager – the person responsible for short-term and long-term growth.” The model they built scaled seamlessly across cities and allowed each manager to make adjustments in the areas that didn’t quite fit. Through this channel, the analytics team continues to touch the most tangible aspects of the business – the number of drivers onboarded, the incentives that are run, the amount spent per acquisition channel, and the price of a ride – every single day.
In order to get there, George had to dispel some of the common misconceptions about the role and responsibilities of data and analytics teams. “At many companies, I’ve seen Analytics treated as a reporting or service function. Other teams turn to Analytics for one-off reporting or ad hoc questions, which limits the depth and creativity our work. In my experience, analysts are able to drive the most impact for the business when they can dive deep into the data, which is hard if they’re constantly reacting to the needs of others.”
As the business has matured, the complexity of Lyft’s two-sided ridesharing marketplace has made questions increasingly difficult and their answers more nuanced. Maintaining a high-level of customer service at scale requires scouring an enormous amount of data to answer questions about customers, drivers, and the interactions between them. “We need to know: Are rides clean, timely, smooth, safe, and affordable? What types of programs, incentives, and acquisition channels do we to tap into in order to onboard the best drivers in the most efficient and cost-effective way possible? And how do we do that – not just right now –but tomorrow, next week, and next year?” Answering these questions requires not just labor but focus and imagination – something that tends to elude analysts when they’re stretched too thin.
To that end, George’s team has worked to increase data literacy throughout the company. “We brought in business intelligence tools that allow business users to build charts and dashboards with a point and click interface. We have also held SQL trainings for people who wanted to perform their own analysis.” In the case of the operations teams, this investment has proven especially fruitful. “City managers tend to have time sensitive questions specific to their markets. For example, if I’m the General Manager of Los Angeles, I need to know whether the airport promotions we run at LAX are delivering value to Lyft. In order to find that out, I might have to answer a question like, ‘How many rides happened at the airport last week?’ It’s a straightforward question that should be self-serve for local teams.”
Central to Lyft’s data strategy are systematic, scalable methods for increasing data consumption throughout the company, which allow analysts to work on high-value projects. “We give teams the tools to explore data on their own so that Analytics can be more effective.”
Organize (cross-functionally) for impact
George’s second ingredient for an impactful data and analytics function is a supportive organizational structure. Lyft’s cross-functional teams align people across organizations with a shared set of initiatives and incentives to reduce inefficiencies. By working shoulder-to-shoulder with their business partners, analysts develop a richer context and appreciation for the particular problem that their team has been tasked to solve.
“For instance, we have a passenger acquisition team with people from product, engineering, analytics, and marketing. At the end of the day, all of them have the same goal – to bring in new customers for Lyft. So, instead of having to compete with one another to get their organization’s needs met, team members work together to problem-solve.”
The cross-functional structure builds lasting relationships and brings business partners, in turn, closer to the analytics process. “Analysts uncover business opportunities based on the data, product and engineering implement and test the changes, and then marketers build campaigns around them. Because everyone works in close proximity, hiccups in that process are addressed immediately.”
People working in cross-functional teams also share information with one another more frequently, which lessens the need for time-consuming meetings and keeps everyone up to date. “I think people who work together regularly tend to be more open to each other’s recommendations. And business operators who work with technical people understand the data behind those recommendations better, too.”
Most importantly, cross-functional organizations maintain a healthy ratio of product managers to data analysts. “A one-to-one or one-to-two mapping of product managers to data analysts [respectively] prevents analysts from having to juggle competing priorities.” It also leads to better analysis. “Analysts that are able to focus on one specific area of the product become true subject matter experts in their problem space.”
That kind of dedicated focus has a ripple effect, making for more accountable and curious analysts. “People feel and identify with their cross-functional teams. They want their projects to succeed.”
When scaled company-wide, the organizational structure gives analysts the time, tools, and tenacity to pay attention to detail. And when it comes to solving for something as complicated and qualitative as great customer service, detail reigns. “When Lyft’s customers open our app, they have expectations. That there will be a driver a few minutes away. That pickup will be seamless. That the car won’t smell. That it won’t be too expensive. That they’ll make it to their meeting on time. That they’ll feel safe. And most importantly, that they’ll want to come back for another ride. On the analytics team, we can’t take any of that for granted.”
Invest holistically in data
Even with the best organizational structure around, though, analysts can only be as good as the data they’re running their analyses on – and the ease and reliability of accessing it. Data management is certainly the less sexy side of analytics, but George has found that it is one of the most valuable areas to invest in early on.
“When I joined, we were sending Excel spreadsheets around over email. Thankfully, we’ve moved beyond that, but it took a tremendous amount of work. We brought in third party tools, rewrote our legacy data model to accommodate new use cases, and scaled our infrastructure to handle data volumes on the order of 100x more. We had to build out a significant data engineering team to make it possible.”
According to George, the willingness to spend on supportive analytics functions like data engineering and business intelligence separates the haves from the have-nots when it comes to building a world-class team of analysts. “Building an analytics team without supportive functions in place is like driving a car on an empty tank – a recipe for disaster. Without scalable infrastructure, queries grind to a halt. Without reliable data logging, analyses turn out inaccurate. And without the proper data visualization software, people waste time running the same analyses over and over again.”
Because Analytics straddles the fence between technical and business teams, it often fills the gap in the absence of strong data infrastructure or self-service BI tools. “Analysts can quickly get boxed into one of two problematic roles: being report monkeys for business teams or being stand-ins for data engineers.” To ensure that analysts remain analysts, the data and analytics teams have to be properly set-up before they are built out.
To avoid the analyst-turned-data-engineer trap, “companies need to have a solid data engineering function.” Hiring analysts for their data engineering savvy creates problems in the long-term, says George. “Either the analytics team will build out the infrastructure badly and the data will be unreliable, or they will build it out well but won’t have the skills to perform high-impact analysis once they finish.” Regardless, it’s not an effective use of time or talent. The same is true of the report monkey problem. To prevent it, analytics has to be made accessible to non-technical employees.
Holistic data education combined with proper data infrastructure can significantly reduce analyst busy work and help data and analytics reach the sweet spot at the peak of George’s pyramid. “Analysts need the headspace to think and work creatively.” Constantly fielding ad hoc requests and patching up holes in data infrastructure prevent them from performing the sophisticated creative work that only they can do.
“Our analysts not only want to understand the business but also grow and improve it in a meaningful way.” Instead of digging through the data in hopes of stumbling upon a relevant insights, the data and analytics team considers what kind of analysis would best contribute to building better business practices. In that sense, being an analyst at Lyft goes beyond asking and answering questions. “As a team, we want to have impact. Not just on the company, but on the world.”
Scale a data-driven culture
At Lyft, it’s not just analysts who care about using data to make better decisions. In fact, data has always had a seat at the table, largely due to Lyft’s founders. Given the array of complex problems posed by Lyft’s two-sided marketplace, co-founders Logan Green and John Zimmer knew from the outset that the team’s capacity to collect, understand, and act upon Lyft’s data would determine the company’s success or failure. George sees the continued impact of that realization across functions at Lyft, even as the company’s headcount has exceeded 1,000. “Don’t underestimate the importance of executive support. Culturally, emphasis on data and analytics has the best results when it comes from the top-down.”
Of course, support from the top must be actionable to be effective. “Business teams should have well-defined metrics and measurable goals. At Lyft we use OKRs that cascade downward – from overall company goals to organizational goals to team goals.”
Building a data-driven culture is not just the responsibility of executives, however; as the company grows and decision-making is decentralized, data culture has to scale. To make that possible, George encourages asking questions that hold people accountable. “Decisions should have data behind them. Anyone proposing a change should have a response to a question like, ‘What metric are we trying to move?’ Or, ‘Show me the results of the experiment that proves the value-add of this new feature.’”
George gives the example of new driver sign-on bonuses. “We continually look to the data to decide what criteria makes sense for our incentives. It’s more difficult to pick up customers on a weekday in a suburban neighborhood than in a city center on a weekend night, so we take geographic information into account when deciding whether or not to offer a bonus. Then, we rigorously test new incentives against the ones already in effect to make sure they work as expected and motivate drivers to join us.”
Making data-driven decisions doesn’t always require diving into the weeds. “Data doesn’t have to be the sole or even primary input for a decision. But data is always useful regardless of how much weight it’s assigned.” For example, the decision to launch their ride-sharing service, Lyft Line, was not driven solely by data. “From the beginning, Lyft Line was part of our vision for building more collaborative transportation options and reducing cost. It was the first product of its kind.”
That didn’t mean the team ignored data input when it came to designing and implementing the product vision. “We simulated the economics and ran small-scale employee beta tests. Then, we talked to existing customers to find out what our users wanted out of the product.” Post-launch, George and his team have run countless experiments to fine-tune the experience for customers and drivers. “Today, Lyft Line looks and feels very different from the first version we launched.”
George’s 45-person analytics team combined with Lyft’s strong data engineering function make continued improvement possible. “Working here has made me appreciate just how much data can impact the way people see and approach problems. Because my team has seen firsthand how our recommendations shape the company’s path forward, we tend to be much more bullish in terms of what we think we can achieve.”
The data-driven culture that has provided George’s team with the time and resources to better business practices has also enabled their colleagues to make thousands of critical decisions every week. “Three years ago, we probably had less than 10 people in the company looking at data. Today, we have hundreds.”
And that engagement is growing. The data strategy that put Lyft on track to complete 350 million rides a year has sparked a doubling-down on investments in analytics. Nothing speaks louder to a data-driven culture than headcount, and Lyft plans to bring on forty more analysts in the coming year. In that growth, George sees tremendous potential. “We want to continue raising the bar when it comes to customer experience. Ultimately, we see a world where everyone, everywhere uses Lyft whenever and wherever they’re going.”
The right data strategy helps Lyft get there.