The 3 As of becoming a data-driven organization
Over the past decade, a growing number of organizations have begun fancying themselves “tech companies,” “data-driven,” or even “data-obsessed.” What used to be Silicon Valley jargon now punctuates meetings across the world, from NGOs, to media companies and other more traditional industries.
We’ve all become so hot for data that, today, there are 1 million data scientist job postings on indeed.com. Yet, despite the urgency to switch on the data lightbulb, most organizations are made up of people who aren’t used to dealing with data on a regular basis. And for a company to truly become data-driven, data needs to visibly influence every major decision across departments— from annual OKRs to the smallest A/B tests.
Good data is accurate
It’s simply useless to have numbers that can’t be trusted. While this may seem obvious, it’s something that’s often widely overlooked. In my travels, I’ve found that the two main culprits for inaccuracy are:
- Having multiple data sources and struggling to reconcile inconsistent numbers.
- Humans being humans, not computers (most of us).
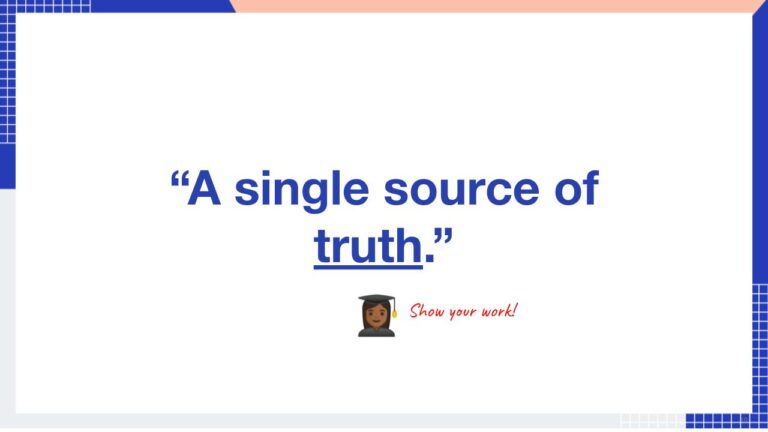
The solution is one we’ve all heard before: having a single source of truth. There are two important steps for achieving this:
- Establishing a common dictionary so that everyone understands each metric to mean the same thing (i.e. what exactly constitutes a “conversion”?).
- Ensuring that the math behind every data point and calculation is shared widely with other stakeholders. Show the work, not just the results (just like we did in school).
Unfortunately, without these steps, what a “single source of truth” often comes to mean is that one person or department owns the data. Yet, the only way to ensure accuracy is to allow other bright minds into the room, and to encourage questioning by the people who orbit closest to those metrics on a daily basis.
Accuracy also means propagating a culture of statistically significant data. Statistical significance is the likelihood that a relationship between two or more variables is caused by some factor other than chance. To show significance, sample size data needs to be large enough. Free sites like abtestguide.com/calc/ are a good starting place for determining whether the data you have means anything beyond a coin toss.
Automation
A second important way to ensure accuracy is via another “A” word (sorry, can’t help it): automation. Humans—no matter how intelligent—make mistakes. A lot of them. And while teams often want to remain nimble and scrappy (does manually uploading data into Excel docs strike a chord?), that’s where tiny mistakes become untraceable steps to incorrect conclusions.
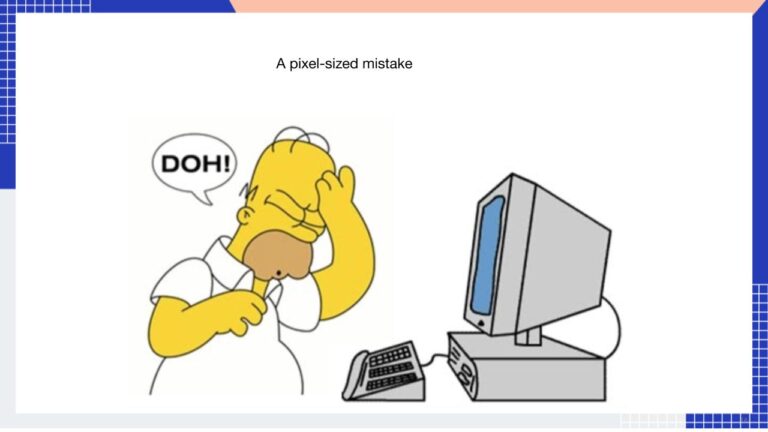
Not only can automation prevent single points of failure (i.e. a distracted human), but it can also be used to trigger alerts for data discrepancies and anomalies—ones that would be extremely difficult for even those who boast “attention to detail” on their resumes to detect.
Good data is accessible
For data to matter, every single stakeholder in the organization needs the ability to pull it, review it and derive insights from it. Simply put, they need access.
But the harder the data is to obtain, or the tighter the bottleneck, the longer it takes teams to arrive at insights.
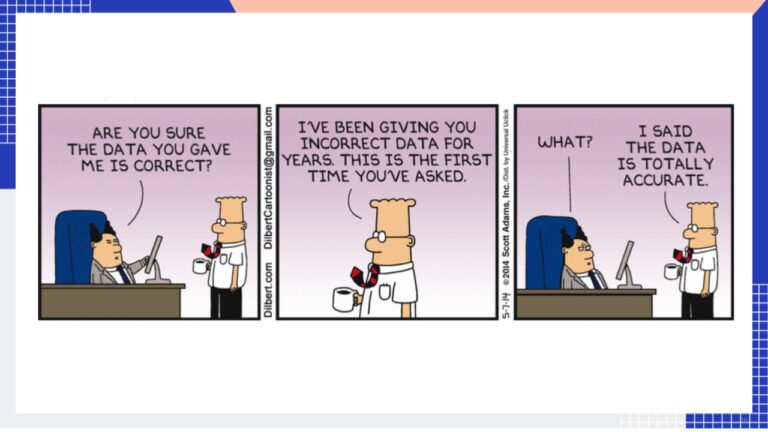
Product and growth leaders will do well to avoid internal silos by including stakeholders in early discussions on data. For instance, Marketing and Sales teams often have important questions that data can answer—make sure tracking capabilities are built with relevant business goals in mind.
Data as a common language
Don’t underestimate the power of language when it comes to demystifying data. For data to be truly accessible, there needs to be an internal, agreed-upon dictionary of terms. Does your team explicitly define what counts as an MQL (Marketing Qualified Lead)? Do the Sales and Marketing teams align on that definition, too? And what counts as a “conversion?” Across your organization, teams should work to build internal alignment on these terms and how they’re used in the business (take for example Airbnb, which even has its own Data University).
Once you have consistency in language, use it. I’m a firm believer that every meeting should reference data.
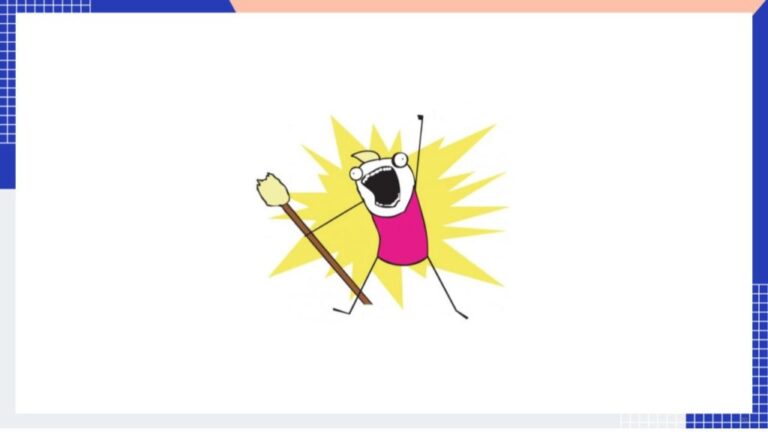
Another important element of “access” is making sure your teams can easily surface the right data. While it might be tempting to pull all the data in sight and sort through it later, what results is unnecessary noise and confusion. Instead of creating situations where your team has to backpedal into analysis based on a mountain of data, be intentional about what you collect at the outset.
Good data is actionable
So, you have some pretty reports with numbers that look good. Now what? Truly data-informed teams recognize the difference between actionable insights and vanity metrics.
Asking “why” when observing any data trend is critical to understanding how to take action. The answer to this question will equip you with the information you need to replicate a positive result (or avoid a negative one).
Challenging your teams to report actionable data discourages people from falling into the trap of self-congratulatory optimism. It also does a much better job of surfacing key findings that can be relied on across the organization. At the end of the day, every decision should be informed by data.
Save the ‘set it and forget it’ philosophy for your Instant Pot, and never set a goal and neglect to track the outcome.
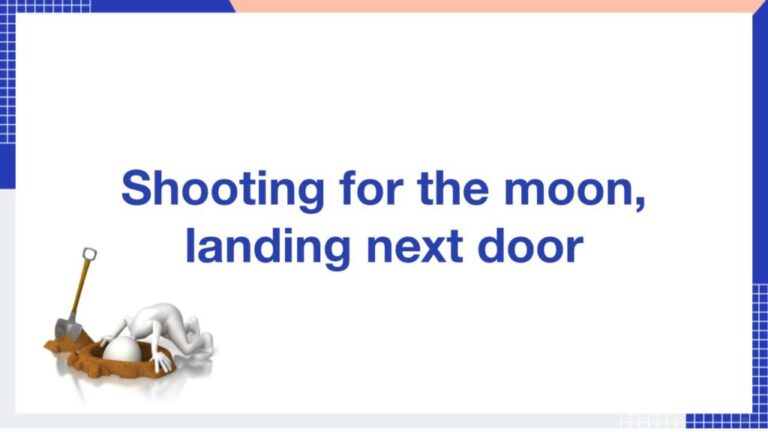
A word of caution in your (conversion) path to enlightenment: beware the line when data moves to the dark side.
Sometimes, being data-obsessed can cause leaders to lose sight of the bigger picture, giving teams tunnel vision when it comes to day-to-day performance metrics. It doesn’t matter if click-through or open rates are through the roof if the increased activity isn’t translating to more sales. Don’t treat metrics and true KPIs (Key Performance Indicators) as one and the same.
Significant results, nimble data
Along with making sure you’re being data-informed and not data-obsessed, it’s important to keep in mind that nobody has enough data to test everything.
When choosing metrics to track and experiments to run, be strategic and go for high-impact categories. Test data-driven hypotheses and take big swings. Running smaller, multi-arm tests only breaks down potential statistical significance and doesn’t always bring us information we can act on.
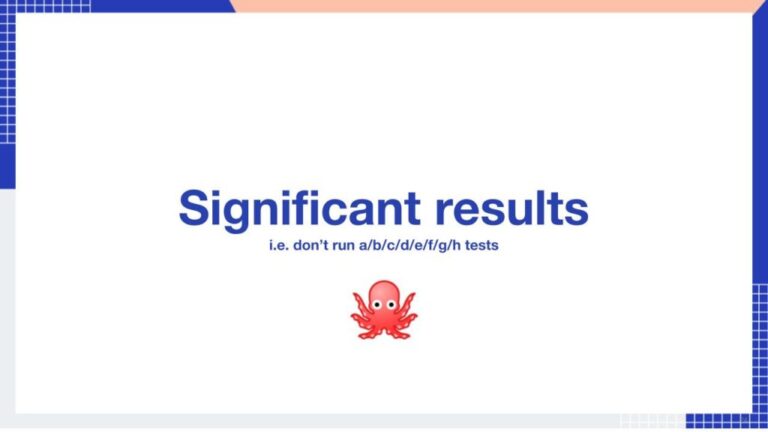
Finally, for data to be actionable, it has to be nimble. If a data question takes days to answer, something needs to be fixed. Could automation be better incorporated in your process? Are you tracking too many things? Making data nimble doesn’t need to mean reinventing the wheel. Great tools already exist—find the right ones and make using them part of your team’s process.
Accurate. Accessible. Actionable.
Three elements that, taken together, will guide you on your path to becoming a data-driven organization.
About Gina Gotthilf
Gina is the VP of growth and community at Quartz. Previously, she lead growth/marketing at Duolingo, which she helped grow from 3 to 200 million users in 5 years with no paid acquisition. Prior to that, she led Tumblr’s growth in Latin America and developed digital marketing campaigns for LVMH. Gina was born in Brazil, studied philosophy and neuroscience at Brandeis University, and had the honor of presenting to former President Obama at the White House.