Data thinking vs. product thinking
Data has fundamentally changed how we design and develop products, but there’s a difference between simply reacting to data points and applying the level of analytic rigor needed to yield meaningful results.
Both data thinking at the micro level and product thinking at the macro level need to be synthesized in order to achieve truly breakthrough design in your digital products.
Here’s how.
Understand the role of data with nuance
There’s an enormous amount of ambiguity when it comes to developing products. UX design, branding, feature-set, nuanced differences in user perspectives, and a million other variables can impact (with varying levels of influence) whether our products get used or ignored.
That makes me anxious just thinking about it, and the reality can be even more harsh. We are constantly in the process of making choices that can make or break whether our teams, projects, and companies are even allowed to exist. So it’s natural to seek better evidence for each and every one of these choices, especially if said evidence is allegedly more objective and less prone to human error.
That’s where data comes in. The more we collect on the various aspects of how products work and are being used, the more of our hunches about those aspects into knowns, or on the flip-side, into invalidated hypotheses. The ultimate outcome should be reality-aligned insights.
But being just “data-driven” in product development won’t get you to where you need to go—not without properly zooming out to understand your data within the context of the products you want to build.
Don’t conflate the micro and the macro
Good data is fundamentally micro in the sense that each piece is collected about an individual phenomenon that is being considered as a slice of a much bigger puzzle that constitutes the product under development. The more precise and specific the phenomenon is defined, the more potentially accurate the data reflects what is being measured. The higher the level of abstraction of the phenomenon, the more complexity it has and the more likely we aren’t accurately measuring what we think we are measuring.
One way of understanding this is that there is no single metric that can definitively tell you whether a product will or won’t succeed. The wins are not synonymous. You’d even be hard pressed to find a single metric that can tell you whether you should or shouldn’t pursue an individual feature.
Data thinking can incorrectly point to a product loss
Let’s, for example, imagine you have a mobile fitness app, and you just added the ability for users to chat anonymously with each other as a tab within the app. Moreover, you’ve discovered that 70% of your users are interested in discussing sensitive topics about their health and personal fitness, but lack a low-risk outlet for doing so. So you gave them anonymous chat and positioned it in the app where it can’t possibly go unnoticed.
As such, it seems reasonable that we could measure the success of this feature by tracking the percentage of users that choose to actually open the tab and start chatting—simple, direct, and clear. If they don’t use it, it’s not worth pursuing.
But you’d be wrong.
Sure, if nobody used the chat feature, it’s possible the whole concept truly has no value. But it’s also possible that there are other frictions surrounding the chat feature itself (UI flows, branding, communication of value) keeping users from engaging. The difference between 7% adoption and 70% adoption could have nothing at all to do with the core functionality of the feature, so scrapping it based on early returns from that one metric could prove to be a bad product decision.
There is no single metric that can definitively tell you whether a product will or won’t succeed. The wins are not synonymous.
Data thinking can incorrectly point to a product win
There are so many examples of companies that have deferred to “the data” without considering the big picture, only to have their efforts lead to short-term success, then backfire.
In Lean Analytics, Alistair Croll and Benjamin Yoskovits explain that web analytics company Omniture once discovered that (in the context of online marketing) “scantily clad women generated a far higher clickthrough rate on webpages than other forms of content.” But when their algorithms blindly optimized around this reality, any short-term gain was offset by the fact that company brand was damaged for having utilized this kind of content in the first place.
As they put it quite clearly, “Using data to optimize one part of your business without stepping back and looking at the big picture can be dangerous, even fatal.”
Viewing the data pieces in product context
Once you have the data in hand, you need to think about it. You need to synthesize all the data you can get your hands on into an educated prediction about the next step you and your team should take—which can often be to go get more data!
And that’s product thinking. Yet this step is often overlooked. People will set up their data stack, react like automatons, and then chalk their missteps up to “the data.” What a way to avoid personal responsibility, but that’s also another story.
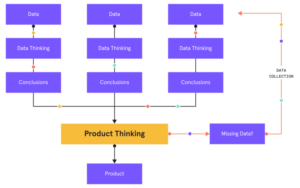
So what does product thinking look like in action?
Do funnel analysis
In the chat feature example earlier, we were tempted to conclude it was a feature not worth pursuing because we had data to show that nobody was using it. However, if we built an event funnel in our product analytics, one that examines what users are doing several steps before potentially reaching the chat feature, we might find that the engagement drop-off was actually happening a screen or two earlier, maybe due to a poorly designed series of buttons or UI flow.
Create counter metrics
Like with the “scantily clad women” branding example earlier, it’s important to make sure any single data win isn’t doing more overall harm than good. One way to keep an eye on this is with counter metrics. If page views are up but so are counter metrics like unsubscribes and customer complaints, then it’s probably going to be a better product decision to ditch the “clickbait” and the empty metrics it’s bringing in.
Pulling it together
Never before has there been better access to rich and nuanced data about the ways your users engage with your products. Today, Mixpanel is but one potential tool to add to your modern data stack, which all together can provide new levels of clarity and stability in potentially every decision you make—but only when it’s used appropriately and in alignment with big-picture vision and goals.
Ensuring that your micro data thinking and macro product thinking remain in firm handshake is the best way to avoid getting overwhelmed, overdiscouraged, or overdelighted by your user data outcomes. What follows will be the best possible versions of your products.
About Joseph Pacheco
Joseph is the founder of App Boss, a knowledge source for idea people (with little or no tech background) to turn their apps into viable businesses. He’s been developing apps for almost as long as the App Store has existed—wearing every hat from full-time engineer to product manager, UX designer, founder, content creator, and technical co-founder. He’s also given technical interviews to 1,400 software engineers who have gone on to accept roles at Apple, Dropbox, Yelp, and other major Bay Area firms.