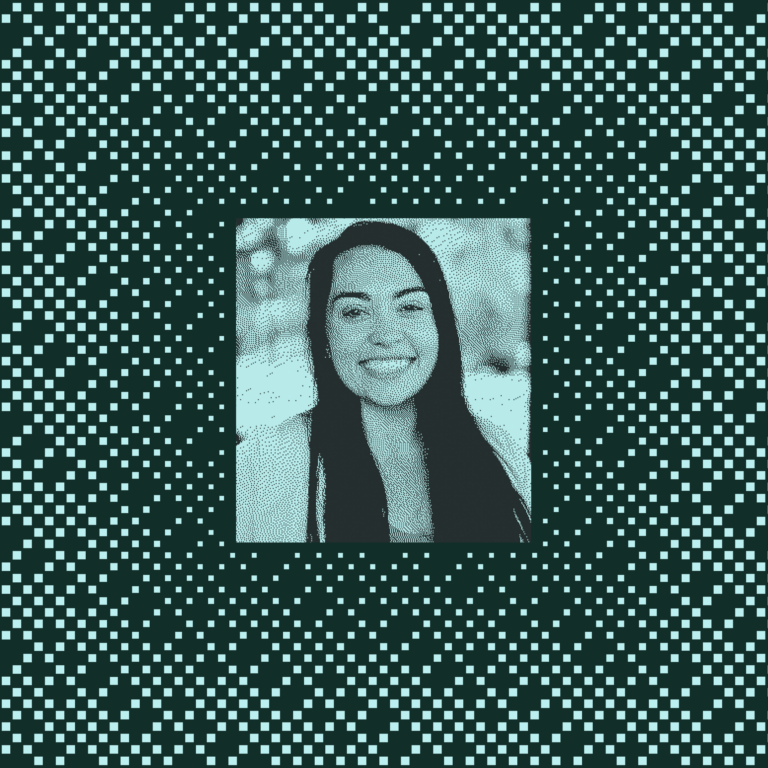
Emilie Schario on making all your hires a ‘data hire’
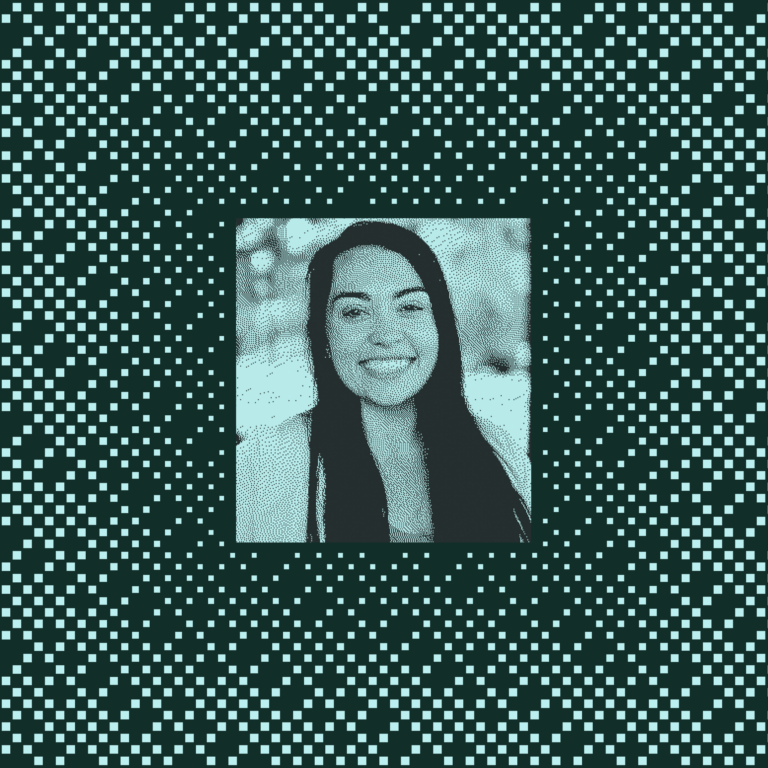
Can you tell us a bit about your journey from a data role to a business role and then back into the data space to start your own company?
It all began when I discovered dbt, an open-source data tool, in 2016. I leveraged this experience to join GitLab's data team in 2018. As GitLab grew, so did my role, spanning from analytics engineer to chief of staff. I noticed that my data knowledge was a superpower, enabling me to provide quick, actionable insights—especially in cross-functional meetings. This realization shaped my perspective on how data can drive business impact.
You mentioned that being in non-data roles actually made you a better data practitioner. Can you elaborate on that?
Absolutely. During my time in non-data roles at GitLab, I gained a broader perspective on business needs and challenges. I realized that perfect precision isn't always necessary; directionally accurate information, delivered quickly, can be far more valuable for decision-making.
I remember sitting in a Zoom meeting soon after Covid started. It was a time of mass layoffs in the industry, so we were talking about how we were going to handle our hiring with so many incoming applications. Someone on the call asked how many applications we were getting a week at the time. In a lot of those kind of meetings, answers to data questions are often, “We’ll have to get back to you.” But I was able to open Snowflake, write a query, and give an answer a minute and a half later. Was it absolutely perfect? Probably not. Was it good enough for us to make this decision right then and move the conversation forward? Absolutely.
"During my time in non-data roles at GitLab, I gained a broader perspective on business needs and challenges. I realized that perfect precision isn't always necessary; directionally accurate information, delivered quickly, can be far more valuable for decision-making."
This experience shifted my focus from complex data infrastructure to the actual impact data can have on the business.
How did your experience at Netlify shape your approach to building and nurturing data teams in particular?
My time at GitLab led to me formulate a number of operational thesis on what made for a great data organization—both from the things that I saw working well and not working well. At Netlify, I had the opportunity to put those theories into practice. That meant if you were someone who cared more about how we set up some fancy infrastructure than you did about what we were going to do with that infrastructure, you probably were not the kind of person I wanted to hire on my team.
I spent way more effort encouraging my team to explore the data freely, fostering a culture of curiosity and discovery.
We created all these different initiatives throughout the org, like giving everyone half a day a week to go swim and float in the data and just find something interesting. You don't have to answer any specific questions, but find something or find nothing—whatever it is, tell us. We coupled this with an internal blog that covered the different discoveries team members made. For example, if someone found that users who were signing up on Tuesdays converted more often, we could share that widely so that it could be enriched by domain expertise. We might then find out marketing was running an onboarding webinar on Tuesdays and that’s where those users were coming from. By surfacing findings, even incomplete findings, regularly, we created a culture of curiosity and letting data drive initiatives.
Too many companies, when it comes to data teams, get so caught up in roadmap and strategic planning and what are the models we have. By focusing completely on impact, we saw firsthand how recentering the conversation led to valuable insights and improved decision-making throughout the company and better support and alignment for the data organization.
You've mentioned the importance of "org-wide curiosity." What does that mean, and how does it relate to the concept of a “data hire”?
Org-wide curiosity means that everyone in your company, regardless of their role, is encouraged to ask questions about the data and seek insights. This doesn't mean everyone needs to be a SQL expert. It's about fostering a mindset where data is seen as a tool for understanding and improving the business. A "data hire," in this context, is anyone who embodies this curiosity and actively seeks to leverage data for decision-making.
"If you were someone who cared more about how we set up some fancy infrastructure than you did about what we were going to do with that infrastructure, you probably were not the kind of person I wanted to hire on my team."
How do you foster this culture of curiosity and data-driven decision-making, especially in teams that aren't traditionally data-focused?
It starts with making data accessible and encouraging questions.
At Turbine, our values of results, ownership, and iteration reinforced the importance of understanding customer needs through data, whether that's quantitative metrics or qualitative feedback. And I’ve carried these values over to my team at Settle.
For example, in order to drive results for a customer, you have to understand and take ownership of the problem you're solving for them and the data that you're working with, which can be customer feedback, notes, call snippets, and things like that. All of those are very important pieces of data, too.
I also believe in breaking down silos between data teams and other functions, like UX research, to ensure a holistic understanding of the customer.
What role do you see AI and tools like LLMs playing in making data more accessible and empowering non-data folks to become "data hires"?
Tools like LLMs have the potential to democratize data access. By translating natural language queries into SQL or other data operations, they enable anyone to explore data without needing deep technical expertise.
A couple of years ago, I wrote this email that basically argued knowing SQL is the new typing. Not that long ago, companies had typists who did all the typing for everyone else in the company. Think of Mad Men: It was rare that you’d see Don Draper sitting down at a typewriter, and it was never the expectation that he was good at it. Today, data folks in a lot of orgs are seen almost like these typists. They're the translators being asked to turn someone else’s question into a data query and then go fetch the answer. So with companies adopting LLMs to help turn English into data queries, we’re working to get past that.
"Not that long ago, companies had typists who did all the typing for everyone else in the company. Think of Mad Men: It was rare that you’d see Don Draper sitting down at a typewriter, and it was never the expectation that he was good at it. Today, data folks in a lot of orgs are seen almost like these typists."
This aligns perfectly with the concept of org-wide curiosity, allowing everyone to contribute to data-driven decision-making.
Any final thoughts on the importance of data hires in today's business landscape?
In today's world, data is the lifeblood of any successful company. Embracing a culture of data-informed decision-making, where everyone is empowered to ask questions and seek insights, is no longer optional. It's essential for staying competitive and driving growth.
Remember, a data hire isn't just a job title; it's a mindset.
I don't want it to be part of the VP of marketing’s job to spend 40 minutes every day building data queries to ask how many people visited our website yesterday. But I do want them to continually think up questions like that—and beyond. So tooling is helping make this a reality.
This means now by just fostering curiosity, you can build a company where everyone contributes to a data-informed future.
This interview has been edited for clarity and brevity.