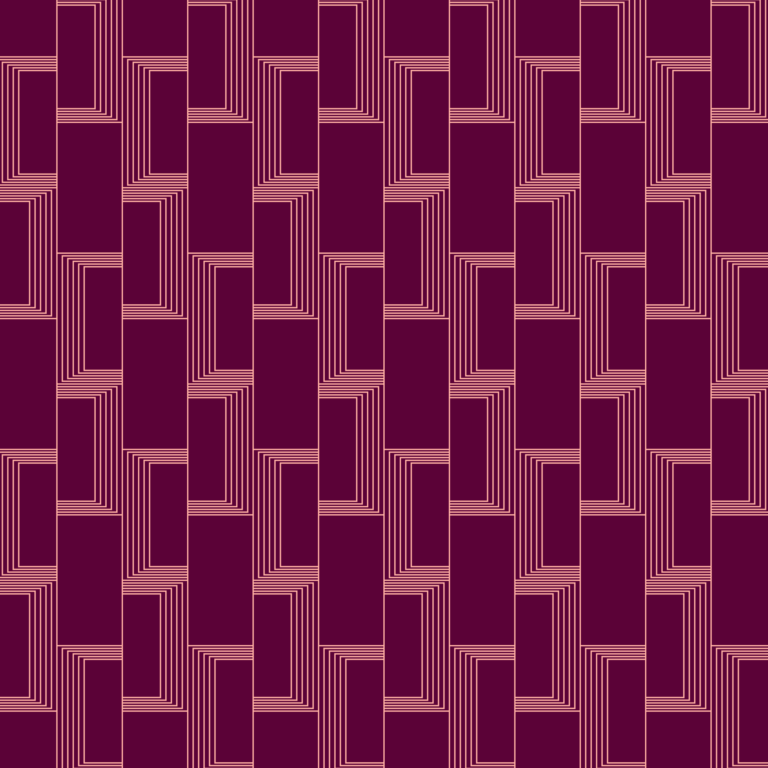
The evolving role of analytics teams: From SQL slingers to strategic thinkers
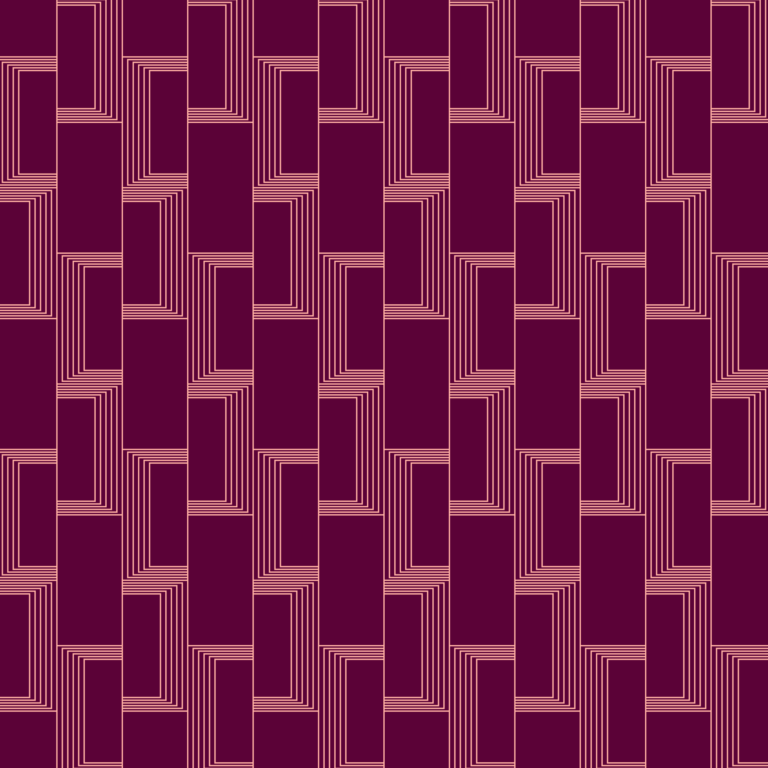
Product analytics roles are changing.
Remember the days when an analyst's primary value lay in their ability to expertly extract and model data using SQL? While those skills remain foundational, the increasing sophistication of self-serve product analytics platforms like Mixpanel, and even some BI solutions, is democratizing data access. Tools are becoming incredibly adept at quickly visualizing large datasets, meaning that the gatekeeping function of SQL for basic product insights is rapidly diminishing. And unless you haven’t been keeping up with the trends in the software, you know that generative AI is only going to further automate so much more of this.
Ultimately, this evolution is a positive one. Instead of dedicating countless hours to writing code that delivers a specific, often tactical, report to a stakeholder, the time of data analysts and scientists can be better spent tackling complex business problems that truly leverage their unique blend of statistical acumen, business understanding, product intuition, and data expertise.
So, if we in product analytics aspire to focus on this higher-value work, how can we actively accelerate this shift?
As a product analytics leader at SeatGeek, I've been deeply engaged in this very challenge. My focus has been on empowering more and more of our product data and product-related data to be readily available in self-service tools. This strategic move aims to liberate our talented analysts and data scientists from what we affectionately (and accurately) call "bs work," allowing them to concentrate on tasks that are not only more challenging but also foster growth in their technical and product strategy competencies.
"Instead of dedicating countless hours to writing code that delivers a specific, often tactical, report to a stakeholder, the time of data analysts and scientists can be better spent tackling complex business problems that truly leverage their unique blend of statistical acumen, business understanding, product intuition, and data expertise."
Through this journey, I've gleaned several crucial insights that I believe are valuable for any organization looking to empower self-service analytics and elevate the role of their product analytics team.
The imperative of proper data alignment
One of the absolute non-negotiables for successful self-service adoption is the ability to align your data properly between source pipes so that your numbers and metrics match—within a reasonable margin of error—across different self-service platforms. It's almost inevitable that some discrepancies will exist due to factors like front-end tracking loss or differences between streamed and batched upstream processes. However, significant discrepancies between reported metrics will swiftly erode user trust and can completely derail your self-service efforts. Investing in robust data pipelines and clear communication around potential data limitations is paramount.
This is an area where Mixpanel's Warehouse Connectors has been crucial. Hydrating our streamed events with important business context allows us to filter and slice data in Mixpanel just as we do in every other number-bearing surface in the company. This way folks can do more in the self-service tool and expect it to be consistent, even when the required data lives outside of the event stream.
Data documentation: The unsung hero of self-service
Data documentation isn't glamorous, but it's the bedrock of a successful self-service analytics environment. Consider a seemingly simple event like item:click
. What exactly constitutes this event? What are the possible values for the accompanying item_type
property? Too often, the answers to these fundamental questions reside primarily within the minds (and SQL queries) of your seasoned data professionals.
However, users of a self-service platform lack this implicit knowledge. At best, they will inundate your analysts with basic inquiries (a clear form of process waste), and at worst, they will generate insights based on flawed assumptions about what a particular product action truly represents. To mitigate this, a significant investment in comprehensive data catalogs—like the Lexicon within Mixpanel—with clear and concise descriptions of events and properties is crucial. Furthermore, you need to cultivate an organizational expectation that self-service users actively utilize these resources. While you'll likely still encounter questions (we even have a dedicated Slack channel for them at SeatGeek!), reinforcing this expectation can prevent non-data stakeholders from drawing erroneous conclusions based on a misunderstanding of the underlying tracked actions.
"Hydrating our streamed events with important business context allows us to filter and slice data in Mixpanel... This way folks can do more in the self-service tool and expect it to be consistent, even when the required data lives outside of the event stream."
Setting clear boundaries: What's self-service and what's not
While the goal is to empower stakeholders with self-service capabilities for a significant portion of their analytical needs, it's unrealistic to believe that every potentially interesting data point will be available at high speed through such a tool. Certain information, such as more complex transactional data, might reside in different systems or formats that are inherently incompatible with your primary product analytics platform.
Therefore, it's vital to clearly communicate what data is accessible within the self-service environment and what requires engagement with the analytics team. This transparency serves two key purposes: First, it prevents stakeholders from wasting their time attempting to find data that simply isn't there. Second, it clarifies when it's necessary to involve analytics and, over time, helps the analytics team identify which additional data sources would provide the most value if enabled for self-service access.
A brighter future for product analytics (and analysts)
I've observed discussions within the data community where practitioners view technological advancements, particularly in AI, as a threat to their job security. Similarly, some marketing materials from BI and product analytics vendors might suggest a future with significantly reduced analytics headcount.
While it's true that advances in tooling will undoubtedly reduce the demand for some of the most repetitive and low-value tasks within product analytics, I firmly believe that this evolution will ultimately lead to a far more engaging and impactful role for those who embrace these new tools. By freeing us from the drudgery of basic data wrangling and reporting, these advancements will allow product analysts to truly leverage their "unique toolkit"—their strategic thinking, their ability to translate data into actionable product insights, and their deep understanding of the business.
As the MLB season’s been heating up, the extra bandwidth created by Mixpanel and other self-service tools has been huge for my team. We’ve been able to spend our time on causal inference studies to help us understand things like the business opportunities that may come from improving images on our site or the effect of default sort orders on buying decisions. We still, of course, field one-off questions from curious stakeholders, but the embrace of self-serve by our product development org has had a meaningful impact on our ability to focus on this more in-depth work.
"[My team has] been able to spend our time on causal inference studies to help us understand things like the business opportunities that may come from improving images on our site or the effect of default sort orders on buying decisions."
The future of product analytics isn't about being replaced by technology; it's about being empowered by it to do more meaningful and impactful work. It's about moving beyond being SQL slingers and becoming true strategic partners in the product development process. And that's a future I'm genuinely excited about.