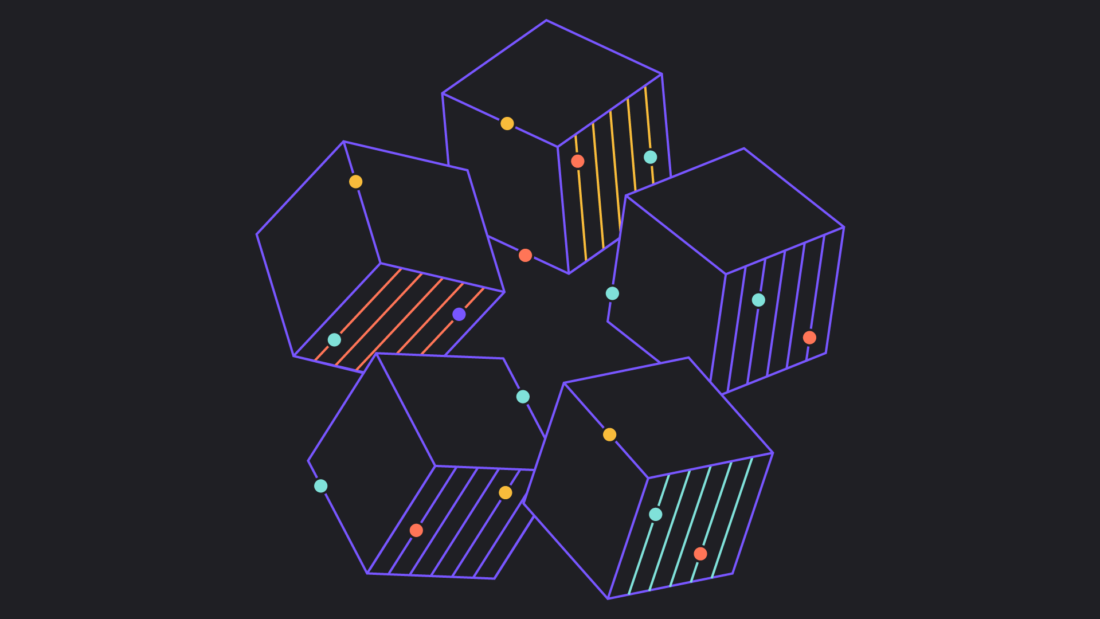
I’ve helped build 5 startups: Here’s how product analytics cuts costs and headaches

For startups, product analytics isn’t just a “nice to have” tool in your stack of tech solutions that are supposed to help you grow. In my experience, it can sharpen your whole approach to product development and engineering.
Put another way: I wanted to call this article “How to save money, brain cells, your engineering/product relationship, and the grand vision of your startup.” We went a different direction, for obvious reasons, but hopefully that bit of unsolicited honesty gives you an idea of where we’re headed.
Like most everyone else in SaaS, I know that product analytics are helpful for growth—that’s why I started using Mixpanel five startups ago. But as I’ve used the tool, I’ve realized that it’s a lot more than that, especially for resource-light startups. This article digs into how to use product analytics as the core of your startup’s operation.
My hope was to publish something that would’ve been helpful to me back when I was just starting out. And my hope is that it will help you, too.
Product analytics can help in more ways than you think
You know this: Startups are almost always resource-constrained, demanding calculated care to work on the right problem in the right way so that no drop of energy is wasted. So you can imagine my delight when it became apparent to me that applying event tracking for just about every key product indicator (KPI) I put together could create a whole new world of not just product analytics, but operations analytics.
See, as an engineering leader, one of my core duties is to make sure projects delivered by product departments are executable within the time constraints of the business. Often, a product department will deliver features requests within a project that may exceed those constraints. Product analytics have been indispensable in the planning phases to identify existing, potentially similar product features. From there, it’s about analyzing their real-world usage to determine whether engineering resources would be best deployed for a new, potentially time-consuming feature or whether that feature needs to go back to product to optimize or remove.
In every high-performing startup I’ve been part of, there has been a healthy data-based relationship between product departments thinking of all the amazing things we could build and engineering determining whether those amazing things can be created within a time frame that suits the goals of the company as a whole (that’s the positive way of putting it, anyway).
Product analytics just puts everyone on the same page.
When you’re a resource-strapped startup, using data to back up your product and operations decisions can be a lifesaver. Your decisions not only become easier to make, they also have a better chance of buying you extra runway to make plenty more.
Before we get into the big picture of using product analytics to put the right building blocks in the right place, let’s start with a story.
As I built Kast—a live streaming hangout spot to share videos, movies, games, and live content in a watch party format—we knew that social engagement was key. We built a “groups” feature to collect multiple watch party sessions into one place. The idea was to build discoverability into the platform, but it didn’t seem to be working.
We had an inkling that the feature wasn’t worth it; originally, it was more like jumping into a Zoom call and then having to determine which breakout room you’re supposed to be in. Product analytics made it easy to determine how groups were being used, and we quickly determined that they weren’t being used at all. Most groups had just one watch party—and if a group had multiple watch parties, user drop-off was much higher, which was strong evidence of a paradox of choice.
This level of insight gave us what we needed to convince everyone involved that it was best to nix this feature quickly, which immediately solved a friction problem and increased usage by decreasing the time to both set up a watch party and discover watch parties.
When you’re a resource-strapped startup, using data to back up your product and operations decisions can be a lifesaver. Your decisions not only become easier to make, they also have a better chance of buying you extra runway to make plenty more.
That’s why I continue to use product analytics at Riven. We use Mixpanel to identify general usage patterns, and we’ll leverage the tool more heavily as development and product processes demand validation of feature usage for a better experience within the web app.
How to get the most out of product analytics at the startup stage
I’m not in the business of categorical statements. The way you use product analytics—let alone how you make decisions, prioritize your roadmap, or validate new features—will depend on your company, your customers, your engineers… and a whole lot more we can’t get into here.
But I am in the business of building products. And building teams that build products.
Instead of stopping short at “use product analytics” (you should) or “it’ll make your life 10x easier” (it will), I can share the three mindset shifts that helped me make the most out of product analytics.
These aren’t rules or workflows—instead, they make up a kind of distilled philosophy. They’re how I approach product analytics as a mindset, not a software category.
Expand your understanding of ‘ownership’
Product analytics doesn’t belong to just one role, or even just one team. Everyone should take ownership across any team in the organization that has product stakeholders.
I say this for two reasons:
- Tracking more events doesn’t cost anything, but it can mean the difference between informed decisions and stabbing in the dark.
- When multiple teams (and product teams, in particular) are let in on analytical insights, engineering (and its critical-but-pricier headcount) can be better directed to build leaner and smarter.
The benefit of a product analytics tool is that setting multiple teams up for usage-based insights requires little scaffolding. You don’t have to know exactly what you’re looking for in order to determine which events to track. And you don’t need specialized knowledge to start driving those business intelligence (BI) insights. At Kast, it was an individual contributor on the Product team who went in and created BI reports, for example.
On top of the business insights, product analytics also make it easier for engineering teams to break up monolithic releases into a more iterative approach with specific requirements. With every engineer I work with, I try to impress the idea that analytics is part of the ownership of any specific product feature.
In a phrase: Empowering engineers with the usage data for the stuff they’re working on makes them better at their jobs over time. I stand by that whether it’s a robust product engineering team in an office or two technical founders sending Slack messages from the couch.
Caveats here: I say “everyone” should participate in product analytics, but every company will reach its “too many cooks” limit at a different point. I do think it’s better to err on the side of giving all teams a seat at the table. From there, just be sure you’re enforcing strict naming conventions for tracking events so things don’t get lost in translation as multiple teams developing for multiple client platforms dig into product analytics.
Hard data is a good alternative to the ‘fail fast’ mindset
The “move fast and break things” mentality of Silicon Valley often goes hand in hand with running on gut feeling. But validating your hunches with real data—as we did with Kast—will speed up decision making by dramatically shortening feedback loops.
Don’t get me wrong, “fail fast” is still a good philosophy. But if you have the data available, you can make better decisions faster—and you don’t even have to fail in the first place.
Put another way…
- Without data: If we’re going to do it, let’s do it fast and hope for the best.
- With data: Let’s look at current usage to see whether it’s actually going to work or not.
In my experience, the investment in tracking attributes is far less than a quarter of what it would cost to build a feature and then have to trash it later. You could spend three months circling around an idea, or you could validate it immediately and build smarter.
It gets better, too: In the future, predictive elements in product analytics will go further and become even more important for weeding out risky experimentation at startups. (At least, fingers crossed, I have no reason to believe they won’t.)
Supercharge your KPIs with deep product metrics
Founder goals tend to take shape less as goals and more as grand visions for world domination. But the smartest among us know that any plan to conquer the world must follow a plan to conquer the town, which is preceded by a plan to conquer the neighborhood.
Of course, this step-by-step strategy plays out in product work through KPIs. And since startups, especially early on, operate a whole lot in the area of neighborhood conquering, I’ve found that KPIs informed by deep product analytics can be a great way to identify all manner of small wins.
For instance, if you’re not confident an overall user activity surge for your app is possible in the coming quarter (and oftentimes it’s not for early startups), you might be able to find a win by setting screen view goals around a new feature you’re betting on. Are the users you do have finding the parts of your product you want them to? That bit of insight could inspire and encourage an investment forward, whether it be with a retooled approach or not.
A feedback loop for more granular pieces of data keeps priorities in line, adjusts resource usage, and keeps the eventual customer-facing product and source code much cleaner than an approach of bluntly funneling everything toward a North Star metric would.
And the more data you have on your small wins, the easier it is to convince founders, investors, and other stakeholders that the world domination plan is well on track.
Data-driven culture should begin at the startup stage
Most startups are speedboats: They need to get from point A to point B fast and accurately, but luckily they can make quick turns if necessary. (That also means they are easier to flip over and destroy, but we’ve covered that.) Later stage companies tend to be more like aircraft carriers, with more overall power but less of the efficiency and ability to change course.
That’s why, as a company scales, building product analytics into your process becomes more about creating a foundation for data-backed decisions instead of just focusing on quick, one-off validations.
In other words: The core processes behind these strategies have their place in larger, post-startup companies, even if it may take many sound, data-led micro decisions to pull off a single turn with a bigger boat. Getting into an analytics-based mode of operation is a good habit to start when you’re an early company, and the benefits should carry over year after year and growth stage after growth stage.
The bottom line is that every mistake or inefficiency costs a company something. I’ve found that using product analytics to guide your operations from product planning to engineering completion can help you steer clear of a lot of them.
About Tim Flack
Tim is the Director of Cloud Engineering and Infrastructure at Riven. He’s held technical leadership roles at Kast, Wrapify, QuickFire Networks, Givit, and Nine Systems.