BI vs. product analytics: Why you need both
In 2019, industry-leading Business Intelligence (BI) tools, Looker and Tableau, were acquired by Google and Salesforce for over $18 billion combined. More recently in 2020, cloud-based data warehouse Snowflake went public at an eye-popping market capitalization of $70 billion. These massive deals show that BI tools and data warehouses are a powerful combo that companies across the globe are incorporating into their tech stacks. However, they don’t meet everyone’s data needs—particularly product teams’.
BI tools are great at visualizing any data that can be queried from a data warehouse. That means that BI analysis and visualizations can cover a wide swath of data on any facet of your business, whether from Finance, Marketing, Human Resources, or Product. They’re perfect for condensing the huge amount of data in your organization down to a few key metrics or KPIs so that everyone is aware of the same basic facts of your business. But there are two significant drawbacks to BI.
- Because BI is so flexible in the kinds of data that can be visualized, it cannot go as deep as tools purpose-built for a particular domain of analysis.
- It takes an expensive, time-consuming, up-front investment in data to realize the benefits of BI.
The relative strengths and weaknesses can be summarized as follows.
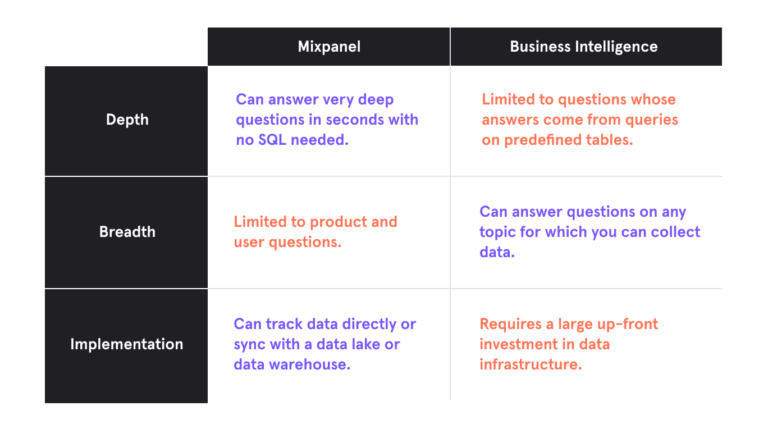
Let’s look at each of these points in turn, and compare them with a product analytics tool like Mixpanel.
Analysis depth vs. breadth
Mixpanel is designed from the ground up to be a self-serve analytics tool for product managers, engineers, and designers to get answers to their questions about user behavior within their products. We use a simple, standardized data model that captures the important aspects of events, users, and other dimensions. That standardization enables us to have a powerful, finely-tuned query engine that can answer questions much more efficiently than a more general BI tool, as well as a purpose-built UI that makes it easy to get answers to even very complex questions within seconds. The end result is that product teams can engage in the rapid, exploratory, creative process of product development with user behavior analysis—without intermediaries and bottlenecks.
To give you an example of the power of Mixpanel’s approach, consider a funnel analysis where you want to see how users progress through your sign-up flow. A multi-step funnel analysis can easily take hundreds of lines of SQL to accomplish, but you can do it in Mixpanel in about 10 clicks. Now, let’s say you want to find out more about the users who drop off at a particular stage of the sign-up flow. In Mixpanel, you can save this group as a cohort and then see if they’re growing or shrinking over time, segment them by any user properties you track, or see the other ways they differ from other users.
All of this is in contrast to a BI tool, where each of these steps would require a new stream of work for the data engineering team to transform the data into the correct format to answer the question. Rather than getting answers to new questions in seconds, it’s more common to wait weeks. Many companies try to plug this hole by hiring dedicated data analysts or data scientists to help product teams query the data warehouse directly to get answers. But because such expertise being in short supply these days, it can still mean waiting around for days for an answer that could have been cracked in seconds.
A product analytics tool like Mixpanel not only gives the answers that product teams need, but it also makes life easier for the data team and the data analysts by allowing them to offload a huge amount of manual work and focus on bigger strategic priorities. We see a lot of our customers find success by using BI to cover broader company metrics, and Mixpanel to enable the product team to go deep.
Implementation
A large up-front investment in data is needed to get value from a BI tool. Most companies that use BI tools have a dedicated data engineering team whose job is to collect data; ensure the quality of the data; transform the data into tables that can be efficiently queried by the BI tool; and load the data into a data warehouse.
Most companies eventually find that they need to make that investment in building their own data stack. It’s the only way to get full control of your data and ensure that all the functions in your company are using high quality, trustworthy data for all of their various needs. But earlier in a company’s lifecycle, it’s not practical to make this investment––and it isn’t truly necessary. On the other hand, product analytics is critical throughout a company’s lifecycle.
All companies, from early-stage startups to Fortune 500 corporations, engage in product development. Rapid feedback on how users are engaging with your product is crucial to finding product-market fit, and also in making already successful products even better. While it would be great to harness BI in the early days, it just isn’t possible for most. Mixpanel can be implemented well before you’re ready to take the plunge on building out a full data stack, but it can also be integrated into your data stack when you do take that plunge.
For companies that don’t have their own data stack, Mixpanel can be implemented quickly by using our own SDKs or a Customer Data Platform (CDP) like Segment or mParticle. This ensures that from day 1 your product teams have access to super powerful product analysis for rapid iteration and growth.
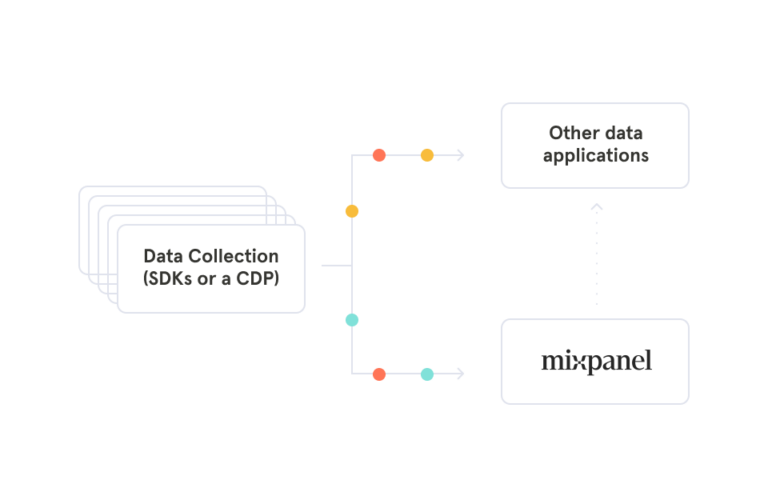
But when you do make that investment in your own data stack, or if you already have, then importing the data from your data warehouse to Mixpanel is the ideal way to integrate. This means that the data that goes into Mixpanel is identical to the data queried by BI tools and other data applications that source data from your data warehouse. The easiest way to import data from your data warehouse to Mixpanel is to use a Reverse ETL tool like Census. Alternatively, you can also use Mixpanel’s Import API.
Integrating Mixpanel with your data warehouse prevents problems that sometimes arise when customers stream event data directly to Mixpanel and also have a data warehouse as their source of truth. For example, say your aim is consistency in all of your data. If an event is tracked client-side with Mixpanel’s SDKs but your data team tracks that event server-side, you can get different answers between Mixpanel and your BI tool. Also, all the work your data team does to improve data quality and enrich events with data from other sources will make Mixpanel even better.
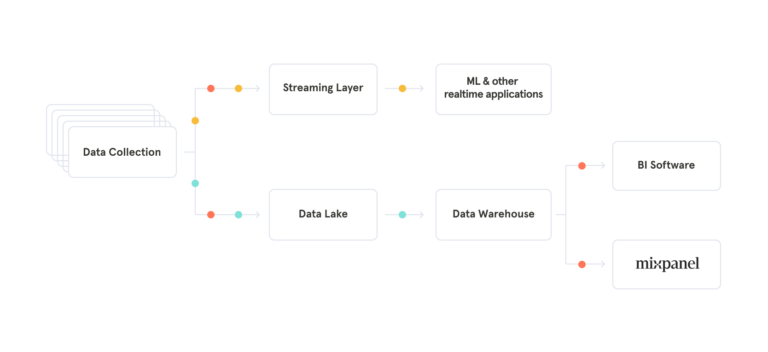
Properly implemented, Mixpanel can serve the needs of your product teams from the earliest days onward and grow with your company. In the end, BI vs Mixpanel isn’t an either/or kind of decision—it’s just a matter of when you’re ready to add BI in addition to Mixpanel. You’re going to need product analytics regardless, and it works great in combination with a BI tool when you’re ready to make a data investment.
Ready to get started? Talk to a specialist today.