A guide to data warehouse analytics for product teams
Data warehouses combine business data from multiple sources like sales, marketing, and customer service into a single repository. Data warehouse analytics is the process and practice of analyzing the combined business data stored in a warehouse to glean insights that will impact strategy and decision-making.
In the last 10 years or so, data has become one of the most valuable business assets that a company can manage. Our ability to collect, store, and analyze data has improved dramatically. As technology has progressed and data warehouses have become more reliable, enterprises have started to use cloud data warehouses as the center of all data operations. These centers combine business data from multiple sources like sales, marketing, product usage, and customer service into a single repository.
Today, fast and accurate analysis is the norm, which is why more and more companies are turning to data warehouse analytics, the process and practice of analyzing the data stored in your warehouse from different sources around the business to glean insights.
Let’s look at how data warehouse analytics works and why it's become so important in modern data operations.
Key advantages of data warehouse analytics
Data warehouse analytics offers several key advantages for digital businesses:
Data centralization
A data warehouse consolidates data from multiple sources into a single organized repository. You can access CRM data, transaction data, and even support ticket data from tools like Stripe, HubSpot, Salesforce, and Zendesk in a single place. You can combine that data to see how your actions in one area impact others.
Without this centralized data source, data silos make it hard for any team to get a full picture of how their actions affect the business.
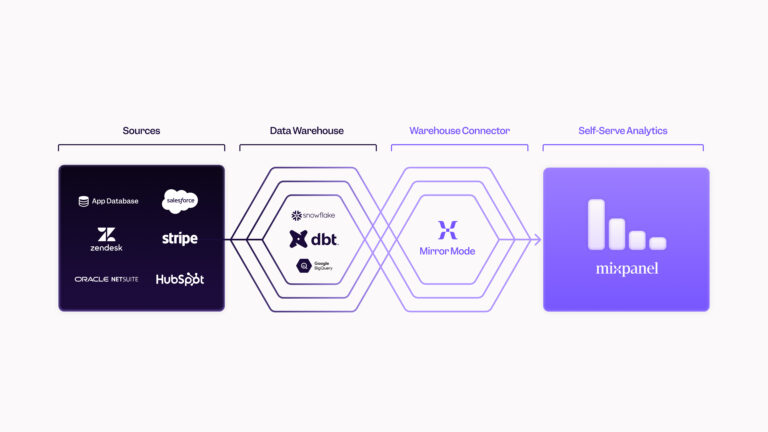
Scalability
Data warehouses are designed to handle vast amounts of data, which makes them invaluable for fast-growing digital companies that have lots of data stored across their tech stack.
Historical analysis
Data warehouses store historical data, which makes it possible to see how things stood at a certain point in time and compare that to how things stand today. This means with warehouse analytics, you can gather deep, long-term insights into things like user behavior or changes in business that go beyond real-time analytics.
Why data warehouse analytics is essential for product teams
Data warehouse analytics can bring together product data (how users interact with your product) with business data like marketing, sales, customer service, and revenue data to create a comprehensive view of company performance that lets you ask more complex questions.
For example, by enriching your product data with warehouse data, it's easy to tie specific business outcomes to feature releases and product updates. With the right tech stack working in tandem, you can answer ad hoc product questions without relying on data analysts to stitch that information together.
Let’s say you ship a new tutorial feature, and you want to know if this tutorial decreased the number of support tickets you receive. If your support ticket and product data are synched, you can answer that question pretty easily.
With the right tool, you can even break it down further and see if people who watch more tutorials are submitting fewer tickets and how those two actions are correlated—which can tell you how effective your tutorials are.
With a well-integrated data warehouse, insights can be easily shared across teams, ensuring that product designers, managers, and developers all have access to the same data.
Data warehouse analytics with Mixpanel
Data warehouse analytics and product analytics are both necessary to build a high-performing product that users will love (and even more importantly, be willing to pay for). But if the data from those tools is siloed, the insights gained will be limited, and subpar integrations risk introducing errors.
That’s why integrating data warehouse analytics with a tool like Mixpanel using Warehouse Connectors has a lot of benefits. Our biggest recent enhancement, Mirror Mode, enables you to automatically sync accurate, holistic warehouse data to enrich Mixpanel analysis and get deeper insights into what’s driving customer impact.
Connected data warehouse analytics in practice: Enriching product analytics with valuable business data
Leading ecommerce and fashion retailer Zalora was a long-time user of Mixpanel’s product analytics when they started using Warehouse Connectors to sync their product and warehouse data. With this update, the Zalora team can now identify high-value segments, pinpoint stagnant users, and take proactive steps to reduce churn.
Read the full story.
Challenges of data warehouse analytics for product teams
Data warehouse analytics is becoming table stakes for product teams, but that doesn’t mean it’s easy. Here are some of the challenges that product teams should watch out for when they’re working with data warehouse analytics:
Data integration complexity
Integrating a data warehouse with a real-time analytics tool like Mixpanel can require engineering support and proper infrastructure. But after the initial integration and setup, you’ll rely less on your engineering and data teams for answers than you did previously.
Maintaining data quality and governance
Data warehouses are only as valuable as the data they store. Accurate, clean, and up-to-date data is crucial to getting actionable insights.
Connected data warehouse analytics in practice: Democratizing data and reducing the data team’s workload
During tax season, Taxfix’s data team was overwhelmed with ad hoc data analysis requests that they struggled to fulfill in time, and the stakeholder teams were sometimes forced to make decisions based on gut feelings rather than data-backed analysis. By connecting their Snowflake warehouse to Mixpanel, they were able to reduce SQL writing significantly and use data to support decision-making.
Read the full story.
Data warehouse analytics with product data is a winning combo
Data warehouse analytics is an important process for collecting and analyzing business data. However, without product usage data, you’ll always have an incomplete picture. Combining both data sources and integrating your tech stack is the key to deeper, more actionable insights.