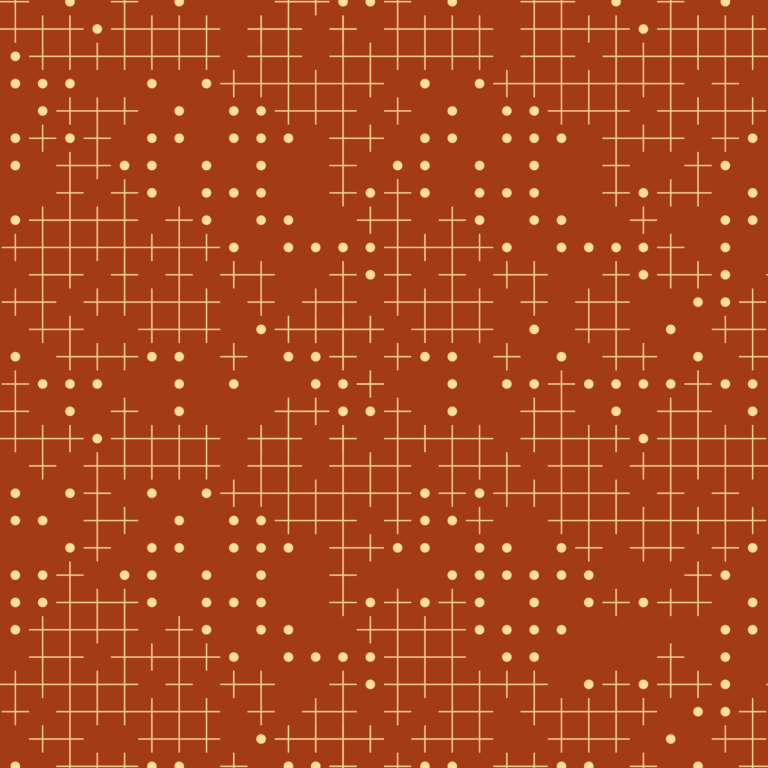
How to find product-market fit with data
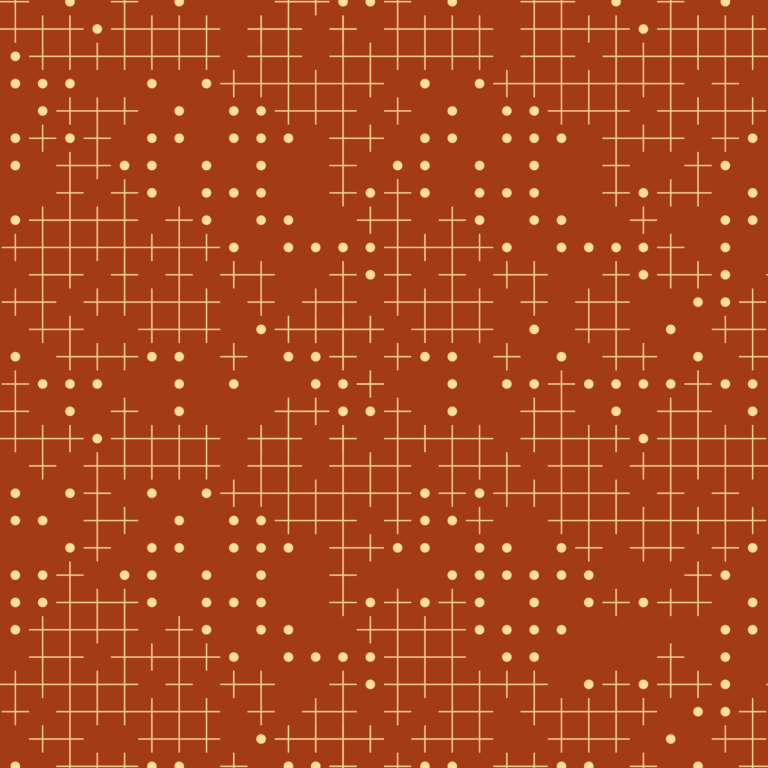
First-time founders know that finding product-market fit is the key to launching a successful company. But they too often assume it’s simple to achieve, especially when building a product they’re excited about.
That’s exactly what it was like for Vlado Hruda, a former Mixpanel PM who ran his own startup for two years.
“Going in, I expected that finding product-market fit would be very simple,” he says. “Instead, it’s like playing a game of Mario–whenever I reached a new benchmark, my princess of product-market fit was always in another castle.”
Finding product-market fit is never straightforward. It’s an ongoing, iterative process, and too many startups run out of funding before they get there (35%, according to CB Insights).
If you want to launch a startup that finds product-market fit and becomes successful, you need to capture insights that will help you build the product your customers want.
And to get those insights, you need data.
The challenges of finding product-market fit
Remember Quibi? The shortform streaming platform closed only six months after launching, despite raising $1.7 billion in funding. People weren’t excited about what the service had to offer—the platform reportedly had only 500,000 paying subscribers when it shut down.
Juicero raised $120 million to create an over-engineered, expensive juicemaker. At $400 a pop for a machine that essentially squeezed packets of pre-cut fruit, people quickly realized they could buy and press the fruit packets themselves.
Product-market fit isn’t about raising money, disrupting the status quo, or even having an innovative idea.
It’s about building something the market needs.
And understanding what the market needs can be tricky. When you launch a startup, you rarely have the resources or access to understand your entire potential customer base. You can only see a small piece of the picture. We imagine that was part of the issue that Juicero faced: They encountered wealthy Silicon Valley entrepreneurs who were willing to spend $400+ on a juicer and mistakenly believed the market for their product was larger than it was in reality.
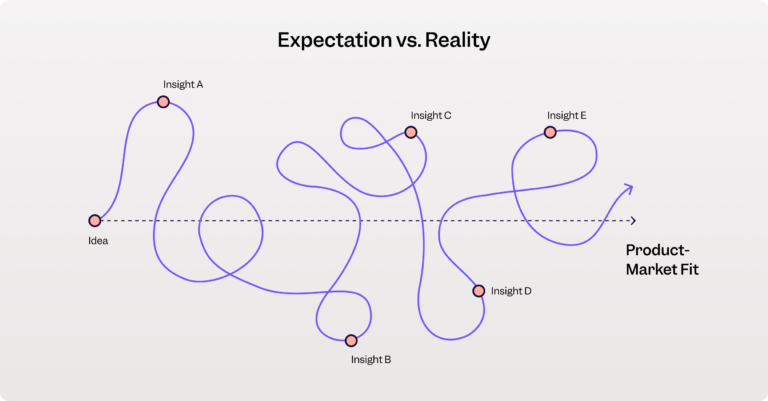
It’s difficult to see your blind spots. Founders are throwing darts in the dark, hoping to hit on the right answers. And even if you do strike the bullseye and get product-market fit, measuring the extent of the fit is harder than it seems.
How to determine product-market fit without product analytics data
To determine product-market fit when you don’t have access to product analytics, you need to gather data by surveying your customers directly. There are two main approaches that you can use.
- The 40% rule
The 40% rule comes from the product market fit survey popularized by Sean Ellis (also known as the Sean Ellis Test). It asks users, “How would you feel if you could no longer use this product?”
There are only three answers to choose from: very disappointed, somewhat disappointed, and not disappointed. According to Sean Ellis, if over 40% of respondents say they would be “very disappointed,” you’ve achieved PMF. - NPS
NPS, or Net Promoter Score, is a survey used to measure customer satisfaction across a variety of industries. It asks, “How likely would you be to recommend this to your family and friends?” A high NPS means you’ve likely achieved PMF.
Both of these methods can help you understand if you’ve achieved PMF (or if you’re getting closer), but they can’t help you optimize PMF or experiment with different hypotheses. To do that, you need to look at user data.
The most effective road to PMF: Using product analytics data to flatten your retention curve
The most effective way to achieve product-market fit is by using product data to improve user retention. If you have product-market fit, you’re going to see customers stick around and use your product in the long term. Your retention curve will flatten.
Here’s an example:
Let’s say 100% of users come in on day one, and within five days, you’ve dropped to 50% active users.
If in 20 days you’re still seeing 50% active users, you’ve got a flat retention curve, which means people are sticking with your product.
Product analytics can help you find the insights you need to flatten that retention curve. Once you’ve hit a flat retention curve, you can experiment to find ways to raise it (to 55 or 60%, in this example). The higher your retention rates, the higher the lifetime value (LTV) of your customers.
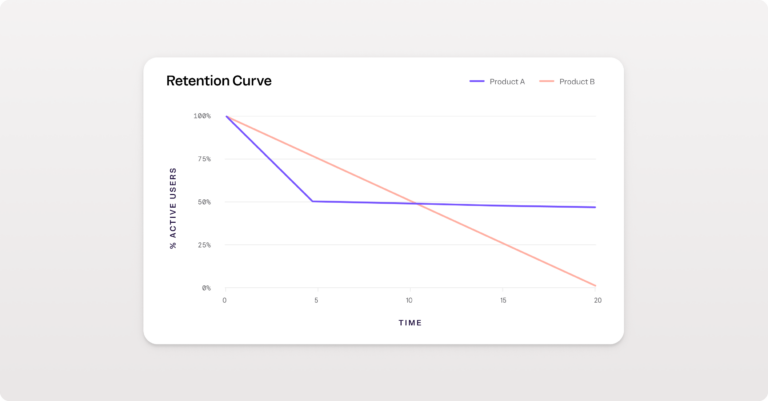
Retention is the best indicator of product-market fit. So, how do you retain users?
You help them build habits around your product.
When a customer first discovers your product, they sign up and begin poking around.
At some point, your user will reach an "aha" moment. They will experience the value your product delivers. The more aha moments they have, the more excited a user will be.
The key to retention is to guide users to reach aha moments earlier and push them to repeat those actions that bring them value. Once a user establishes a habit around the value that your product delivers, they’ve reached a “habit moment,” and you have retained that user. Do that at scale, and you’ve achieved product-market fit. Product data will help you determine aha moments and habit moments. But more than that, it will help you see which changes to your product, onboarding, or UI will push users toward those actions.
Examples of habit moments
Different companies have shared their habit moments. Here are a few examples, from Reforge.
- For Pinterest, the aha moment is pinning a first pin within seven days. The habit moment becomes a habit when a user does this every single week within the first month.
- Slack’s habit moment comes when a user has exchanged at least one message for four out of seven days.
- For Zoom, the habit moment is when a user has hosted four meetings within the first 28 days.
How Mixpanel used data to flatten our retention curve
Here at Mixpanel in the early days, we faced the same challenges that all startups do. We needed to determine our aha moment and help users get value so they would return to our tools again and again.
We used our own platform to gather the data and insights to understand when our users reach value and what makes them become habitual users.
We saw that users’ aha moment comes when they interact with their product data and uncover their own insights in Mixpanel.
We built a funnel to analyze the likelihood of each user gaining insights from their data in Mixpanel and reaching that aha moment.
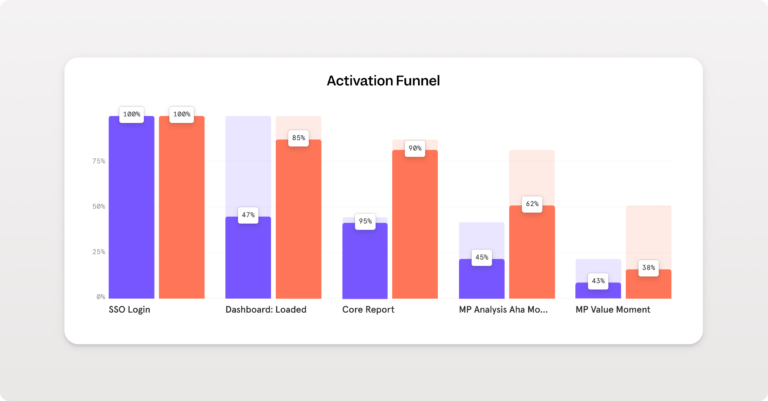
This funnel (above) is for a user who is joining an established project.
By looking at the data, we realized that there’s a lot of friction in doing data analysis by yourself. But if you’re part of a team, you learn a lot from other people’s dashboards and reports. We found that if we nudge these people to look at their teammates’ dashboards, they learn a lot from how their colleagues are interacting with data. They can click into the core reports, play with them, and share new insights with colleagues.
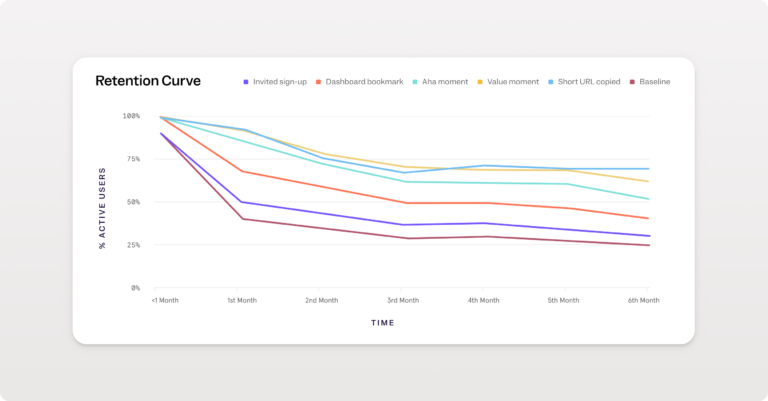
What we found is that for every step of this funnel, users who went further in completing the Invited User Activation Funnel had a higher retention rate—so users who bookmarked a dashboard had higher retention than those who merely signed up, for example.
For those who shared their findings with their teammates (measured by “short URL copied” in the graph), their retention curve flattened out. By nudging more users towards that action, we saw a 3x impact on six-month retention.
For new users joining an established project, the aha moment might be visiting an existing dashboard and understanding how to use the tool. The habit moment is when they share their own insights with their team.
By nudging them towards that action and flattening the retention curve, we’ve achieved product-market fit for these users.
For VC funding, nothing matters more than product-market fit
Product-market fit is one of the most important factors for investors considering whether to fund a startup. Product-market fit is “the only thing that matters,” Marc Andreessen, of VC firm Andreessen Horowitz, famously wrote on his blog in 2007.
Former Mixpanel PM Vlado recalls the same of his own startup experience: “We never achieved product-market fit, and VCs were very reluctant to even talk to us. They have filters on the kind of startups they wanted to fund, and product-market fit was one of those major filters,” he says.
All this is to say: The moment you reach product-market fit, the relationship between you and VCs—and your users—is going to change. Focusing on the right data and metrics is the best way to get there.