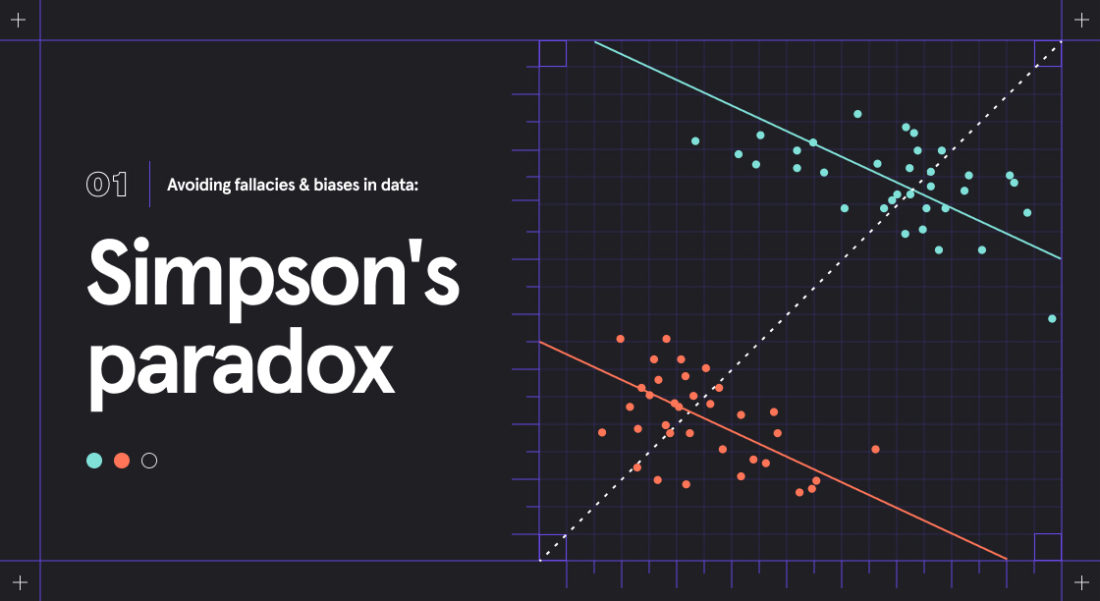
Simpson’s paradox and segmenting data

There’s no debating that data is essential for creating an effective product strategy. And yet, many fail to account for common statistical paradoxes that can result in misleading insights—and have real business consequences. To kick off our series on avoiding fallacies and biases in data, we’re talking about Simpson’s paradox and the importance of segmenting data to distinguish coincidence from fact.
How does (the right) data help inform an effective product strategy?
Crafting an effective product strategy relies on the accuracy and quality of your data, and the insights that result from it. But, as we’ll soon learn in our discussion of Simpson’s paradox, this is tricky if you rely solely on quantitative data.
“Insights can come from anywhere, but most of the time, they come from one or more of four key sources: quantitative insights based on the data, qualitative insights based on interactions with customers, technology insights based on new enabling technologies, and industry insights based on an analysis of the industry trends.” says Marty Cagan, founder of Silicon Valley Product Group (SVPG) and former product leader at companies like Netscape and eBay.
“In my experience, more often than not, the key insights come from the data. So it pays big dividends to spend real time studying it. And in fact, good product leaders often immerse themselves in their data, trying to decide where the real potential is and which of their hunches are worth pursuing,” he adds.
When it comes to crafting an effective product strategy with data, OpenView Ventures Director of Growth, Sam Richard, takes it one step further, stating the importance of analyzing data across the user journey. “It’s so important to collect data on your customer’s journey through your product, especially their journey to conversion and expansion, but also fall offs in that journey as well. I often see scenarios in which analytics focuses more on features and their usage, but not on the individual user experience moving from one classification (‘lead’ to ‘activated’ to ‘power user’) to another,” she says.
But, left unchecked, data can lead you astray
Though integral to any product strategy, too often, data is treated by even the most sophisticated product teams as axioms without further scrutinization of their findings. Numbers can be misleading, and even more so when aggregated. As Mark Twain famously said in his autobiography, “there are three kinds of lies: lies, damned lies, and statistics.”
At Mixpanel, we want to empower everyone to build better products with user insights. But user insights can only be relied upon when underlying trends are cross-examined against common data fallacies and biases. It’s this practice that helps product leaders avoid mistaking coincidental trends for facts.
One such example, and the focus of this piece, is Simpson’s paradox—a phenomenon that not only highlights how trends in your data can be misleading, but also underlines the importance of segmenting your data (so important in fact, it’s one of our primary metrics!).
What is Simpson’s paradox?
Simpson’s paradox, named after a British statistician who first described it in 1951, is a statistical fallacy that occurs when aggregated groups of data show a particular trend, but that trend is reversed or eliminated when the data is de-aggregated (or, simply put, broken down). Understanding and identifying this paradox is extremely important for correctly interpreting data. Otherwise you’re left trying to take action on superficial data trends that don’t really translate into the real world.
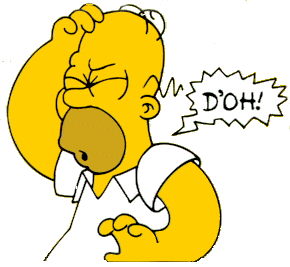
Though Simpson’s paradox isn’t named after the popular TV show, l’ll illustrate this problem with a hypothetical example based on characters from said show (Harvard’s Joe Blitzstein also explains this really well). The show has two characters: Dr. Hibbert and Dr. Nick. While Dr. Hibbert is a prominent and very competent doctor, Dr. Nick is…well he’s a fraud.
In this example, let’s assume that both doctors only do two types of medical procedures: heart surgeries, and band-aid removals. Let’s also assume that in the last year, Dr. Hibbert completed 90 heart surgeries (70 of which were successful) and 10 band-aid removals (all of which were successful). On the other hand, Dr. Nick completed 10 heart surgeries (none of which were successful) and 90 band-aid removals (85 of which were successful).
If we were to run a basic analysis on each doctor’s surgeries, we’d get the following success rates:
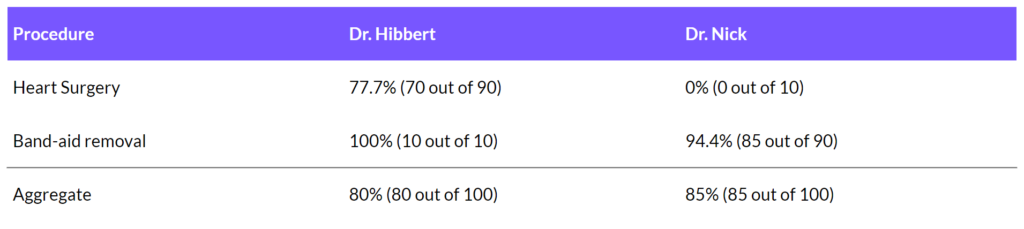
Interesting. Upon aggregation, the data shows that Dr. Nick has a higher overall success rate. But segmenting the data shows that Dr. Hibbert is clearly a better doctor than Dr. Nick for both medical procedures—Dr. Nick is so incompetent that he failed at removing a band-aid. Not once, not twice, but five times!
The aggregation of the two data sets reversed the trends in the constituent data sets and gave us a very misleading insight. However if given the choice to go with one of the two doctors, anyone in their right mind would seek out Dr. Hibbert’s medical services.
A similar example using a mock dataset in Mixpanel can be seen below, where the conversion rate for a simple Funnel report initially shows that users on Android convert better (5.5%) than users on iOS.
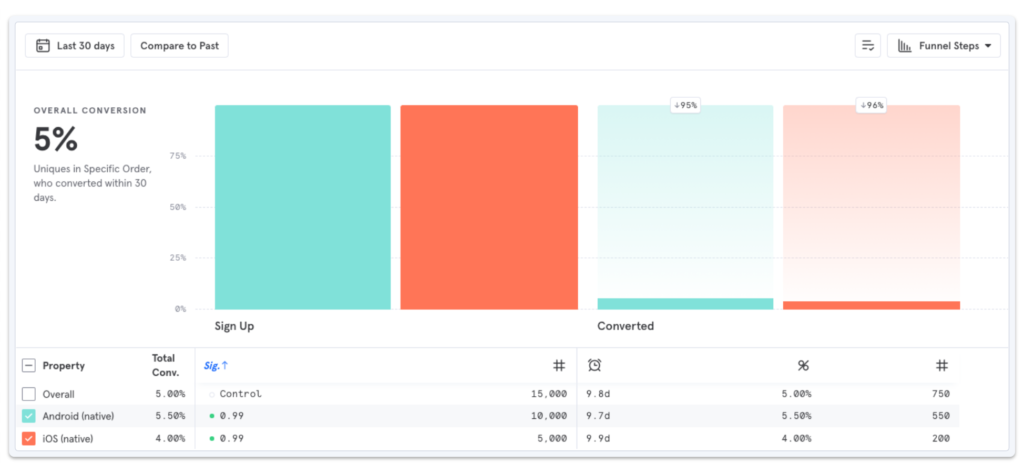
However, segmenting the same data down further by device type shows that users on iOS convert better than users on Android, on both phones and tablets.
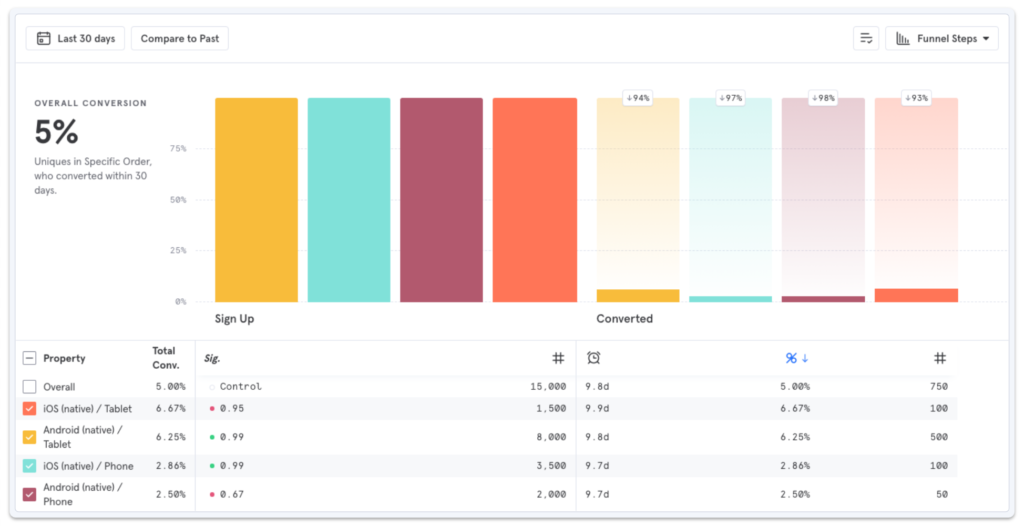
How do I avoid Simpson’s paradox?
The examples above illustrate the limitations of viewing data in aggregate, which might seem unavoidable in today’s data-centric world. How else would you analyze thousands of data sets created by millions of users? Well, the key isn’t to separate each data set, but to segment your data by important factors, or user segments.
“This is a common problem when looking at the entire user base instead of specific segments,” says Practico Analytics’ Ruben Ugarte on Simpson’s paradox. “I was working with a mobile consumer company and was trying to understand why their retention was poor. We were looking primarily at new users in the last 90 days. We then switched to looking at paying users and the most engaged users, and we discovered that retention wasn’t an issue there. The overall trend for the entire user base hid the great retention numbers from smaller groups.”
Finding out which factors matter most also helps you make better sense of your data so you can focus your efforts. In the case of the mobile consumer company, Ruben adds, “Their economic model could work fine with paying users and they didn’t have to spend time trying to fix a phantom problem. Instead, they could focus on replicating what made the paying users great, and acquiring more of those users.”
Despite our best efforts, Simpson’s paradox can rear its ugly head in any type of analysis, which makes avoiding it all the more challenging (and important).
“I always encounter Simpson’s paradox,” says Sam of her work at OpenView. “It can be a churn project, identifying activation indicators for a portfolio company, or understanding which paid channels work for an organization—if I don’t segment the data by things that are relevant to the company, like ideal customer profile, value metric, user role or cohort, I get completely misleading insights.”
How segmenting data can reveal new truths
Not all users are the same, and neither are their attributes and behaviors. As we learned above, finding real insights on why users behave the way they do, and the underlying trends that cause changes in your metrics, is only possible when you segment your data instead of just analyzing aggregates.
But, segmenting data isn’t only necessary to invalidate misleading insights—it can help surface hidden trends that have serious real-world implications too.
Another client that Ruben worked with realized this first hand. They were only looking at the average sign up rate for their product as their success metric. While this number was stable, the quality of users was dropping and they were less likely to complete onboarding or engage with the product. Not finding this out on time would have caused a big hit to overall user engagement that would have likely led to irreparable churn.
“Averages lie, segments (or cohorts) don’t.”
—Ruben Ugarte, Practico Analytics
And when it comes to knowing what makes your users tick, Sam’s thoughts are unequivocal. “This always takes me back to how important it is for SaaS businesses to understand their target buyer segments early—this isn’t an optional exercise—it makes a huge difference in how you slice and understand how different users interact with and find value in your product.”
The takeaway?
Whether you’re analyzing retention data or building funnel reports, don’t look for averages of aggregate data sets. Instead, look for patterns based on important user attributes and segments. Finally, focus on the patterns of the users that matter most (or are most successful) so you can help all your users succeed.
Validate insights from data before turning them into action
Statistical fallacies and biases are a formidable foe that can play tricks on your mind, leading to costly mistakes in data interpretation and analysis. Which, to go back to where we started, is yet another argument for why data should never be used exclusively.
In fact, Marty believes this is often the difference between strong product organizations and the rest.
“We can rarely achieve our goals with just data. The data is essential for telling us what’s happening, or it can help us predict the customer response once we deploy, but it generally can’t tell us why. We need to complement our quantitative understanding with qualitative techniques to help us explain what we’re seeing, so that we can get to work fixing.”
At the end of the day, the best product teams continuously validate their findings with insights from other sources before taking action based on data. It’s a useful process that ensures your insights are reliable, and won’t lead your product down the wrong path (wasting valuable resources in the process).
Ultimately, the best way to prevent data fallacies and biases from ruining your analysis is to know how to spot them in the first place.