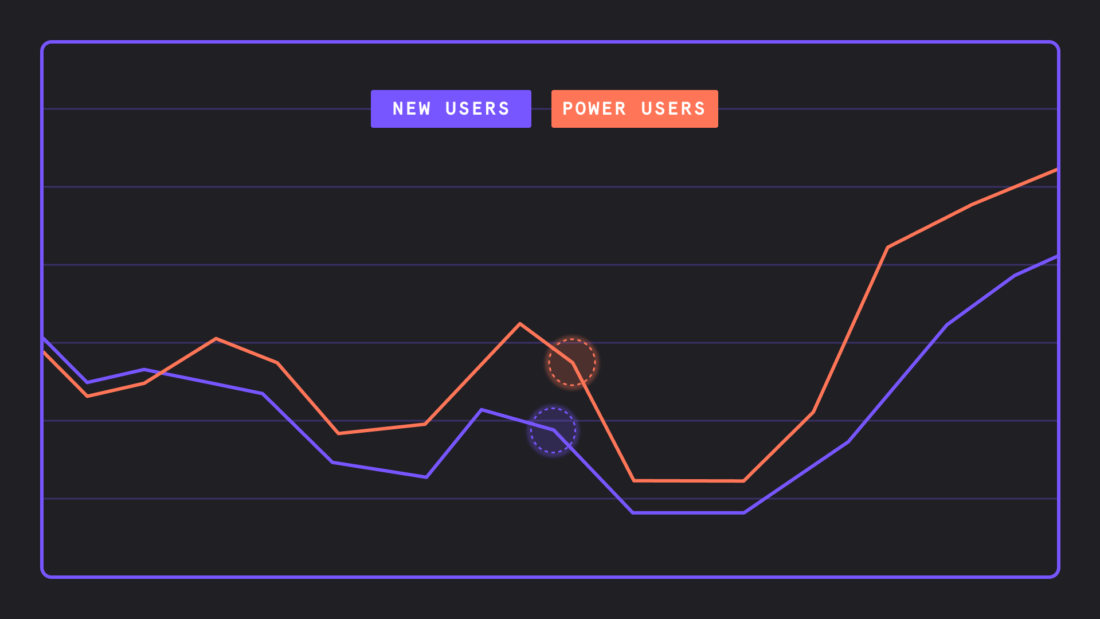
Ultimate Guide to Cohort Analysis: How to reduce churn and strengthen your product retention

Cohort analysis is one of the best ways product analytics can help you both acquire and retain customers. This Guide will take you through cohort analysis, explaining how to reduce churn and strengthen product retention. By digging into actual behavioral data overtime, instead of relying solely on interviews and feedback, you can get a crystal clear picture of a user journey—including where the value moments and roadblocks lay.
With this level of insight, you can both reduce churn and strengthen your overall product. Let’s find out how.
What is cohort analysis?
Cohort analysis is a method for tracking a group of users over time. A cohort is a user group that shares a common characteristic such as an acquisition date, product type, or behavior.
By analyzing a cohort—say, paying users—teams can adjust features of their website or app to cater to those individuals and increase their engagement.
Why is cohort analysis useful?
Customer cohort analysis makes user tracking simpler by grouping users by their behaviors and characteristics.
Cohorts are, simply, user segmentations that are saved and named for quick reference.
Rather than setting new reporting filters each time they log into an analytics suite, teams can name cohorts things like “Power Users,” “Busy Brenda,” or “Week 7,” and check back repeatedly to see how that cohort is performing over time.
Cohorts allow teams to understand their users in a more meaningful way than if they simply looked at data for their entire user base. Broad averages often conceal the specific preferences of smaller groups.
If teams don’t understand and cater to those specific preferences, they can lose those users. Take, for example, a video streaming service that notices that a slim majority of its users—51%—love horror films. They’d make a big mistake by recommending horror flicks to all of their users, some of whom are not interested in horror films and are more likely to churn from the platform if they feel that the service doesn’t cater to them.
With insights into each cohort’s preferences, on the other hand, the video platform could send more relevant recommendations and increase retention for all users.
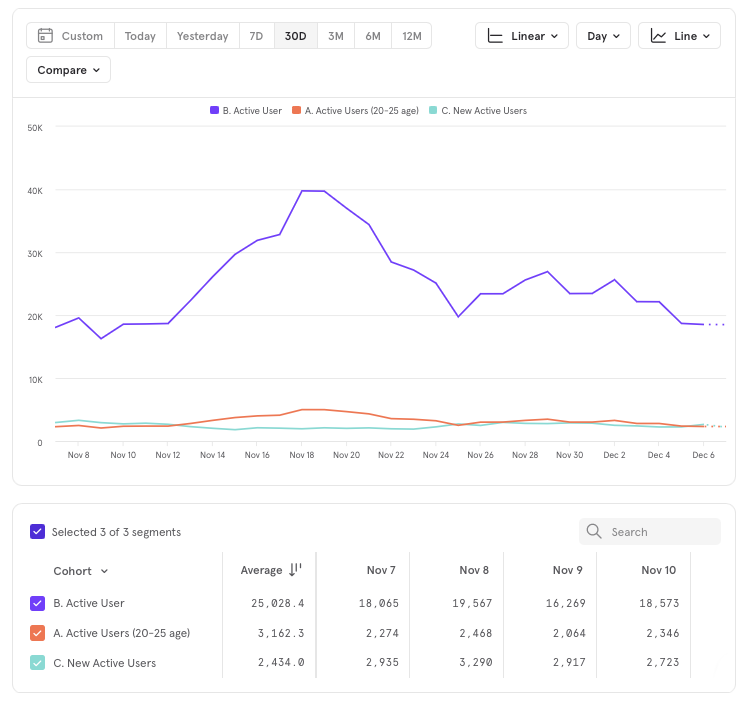
Types of cohorts to consider
Cohorts are defined by common characteristics, experiences, or behaviors—so you can identify patterns of behavior across the user lifecycle.
These characteristics can be, but aren’t limited to:
- Demographic (e.g., age or location)
- Behavioral (e.g., number of times a feature is used or amount of purchases made)
- Technographic (e.g., app or SDK version)
Taken together, you can narrow down your analysis to only those users you want to consider—say, how users aged 21-30 in California are using the app versus the web-based platform.
Common examples of cohorts include:
- Power users, users that made three or more purchases in the last seven days
- Recent upgrades, users that upgraded their plan in the last 30 days
- Inactive users, users that haven’t used your app in the last 14 days
- Acquisition cohort, users that have signed up for the product on the same day or the same week
These cohorts can also include criteria to include or disqualify users with specific characteristics (e.g., use an iPhone or log in on Chrome).
In short, using cohorts provides you with more insights by allowing you to be more particular in analyzing your users instead of relying on broad metrics that may be misleading.
Why you should consider behavioral cohorts
Considering acquisition cohorts and demographic info is good for getting a broad sense of where your product is performing well and how churn trends over time. But you can only prioritize features and take effective action by considering behavioral cohorts (like the power users and inactive users mentioned above).
“When we study user behavior, we gather data on what people do—and what they don’t do—so we can build products that people will value,” says Mixpanel’s Aaron Krivitzky. “After all, you wouldn’t build a product that no one would use, nor would you invest time, money, and energy in a feature set that has no observable demand.”
In our guide to behavioral analytics, we define the process as “sorting and counting behaviors in order to construct behavioral cohorts, and to analyze things like engagement, conversion, and retention—and to measure these things as they change over time.”
In turn, these behavioral cohorts give you the insight you need to both acquire new users and retain users. For example:You can look at which actions power users took early on and optimize onboarding to drive more users to those actions.
Cohort analysis vs segmentation
While plenty of people use ‘segment’ and ‘cohort’ interchangeably, that’s not technically accurate.
Segmenting users in your product analytics tools usually gives you a quick view of a single action—say, the total number of users who have clicked the Upgrade button. Cohorts, in turn, combine events and time periods to ensure you’re looking at the same group of users—users who have signed up this week and clicked the Upgrade button within the first three days, for example.
A cohort, then, is a subset of segmentation—and a critical application of segments in your product analytics.
“Segmentation is the single most impactful thing product teams can do to improve their onboarding experience. And the only way to know that changes in the user onboarding are working is through cohort analysis. It allows you to see how actions users in the cohort took or take translate into changes into activation and retention metrics. Simply put, if you’re not leveraging cohorts in your analysis, you’re most likely just ‘spraying-and-praying’ in improving your onboarding.”
Ramli John – Managing Director at ProductLed Institute
Limitations of cohort analysis in Google Analytics
For teams without a dedicated product analytics tool, Google Analytics is often the default for trying to assess what’s working and what’s not.
While Google Analytics may work for some surface-level insights, it breaks down when teams try to use it for robust segmentation and cohort analysis.
For example, Google Analytics is unable to support the following queries for building out cohorts:
- Did not do “event”
- Did “event” X times across different days
- Aggregate properties (sum, average, distinct count, etc.)
- Event properties where “most frequent” = x
Even more importantly, Google Analytics doesn’t support a multi-criteria cohort breakdown. Instead, retention can only be measured with one criterion. In contrast, Mixpanel can measure retention on two criteria. A few examples of multi-criteria cohorts highlight why this is important across multiple industries:
- How many users came back after “app install” to do “deposit money”?
- How many users that “watch video” came back for “download video”?
- How many users searched your e-commerce site and then came back for checkout?
Finally, you can use Mixpanel’s Frequency report to learn how frequently users return to use your product or service—something Google Analytics doesn’t allow for. Frequency Analysis plays a huge role in understanding how users repeat an action. This can be very useful to understand how often users use a certain feature in a timeframe, giving product managers a sense of where to prioritize features.
How to conduct cohort analysis
To analyze cohorts, teams will need a cohort analytics tool that tracks cohorts with enough informational granularity to be useful.
It isn’t enough to view vanity metrics such as page views or likes, which don’t offer much insight into why users do what they do. Teams need to correlate all user behaviors and characteristics across devices back to concrete metrics such as subscriptions or revenue.
A cohort analytics tool should feature:
- Data ingestion and integrations with common systems like CRM
- Data storage and reporting
- Graphical interface
- Tracking and measurement
1. Select a question to answer
As with any journey, teams must know the destination before they begin.
For instance, is the team most interested to know what factors drive retention, which group of users is most likely to upgrade, or what group of users is mostly likely to commit fraud?
As an example, the team behind a streaming music app could be curious about the users that continue to use its service after 30 days. A cohort could be created to track those users to understand how to attract and retain others like them.
Examples:
- Who are my power users?
- Which users are not opting in to new features?
- How many high-value users use Chrome?
2. Define the metrics
Which metrics help answer the question the team has selected? In the case of the music app, the cohort would include any user that exhibits positive retention after 30 days. However, cohorts can be measured by all sorts of metrics, including:
- Timing, when users signed up
- Behaviors, whether users purchased once or repeatedly
- Characteristics, based on region, age, marketing source
Learn more about the most important user engagement metrics.
3. Define the cohorts
Within the cohort analytics tool, create a new cohort and filter for the behaviors and characteristics that define the cohort in question. Save the cohort and verify that the users gathered within the cohort report match the cohort’s parameters. Adjust if needed.
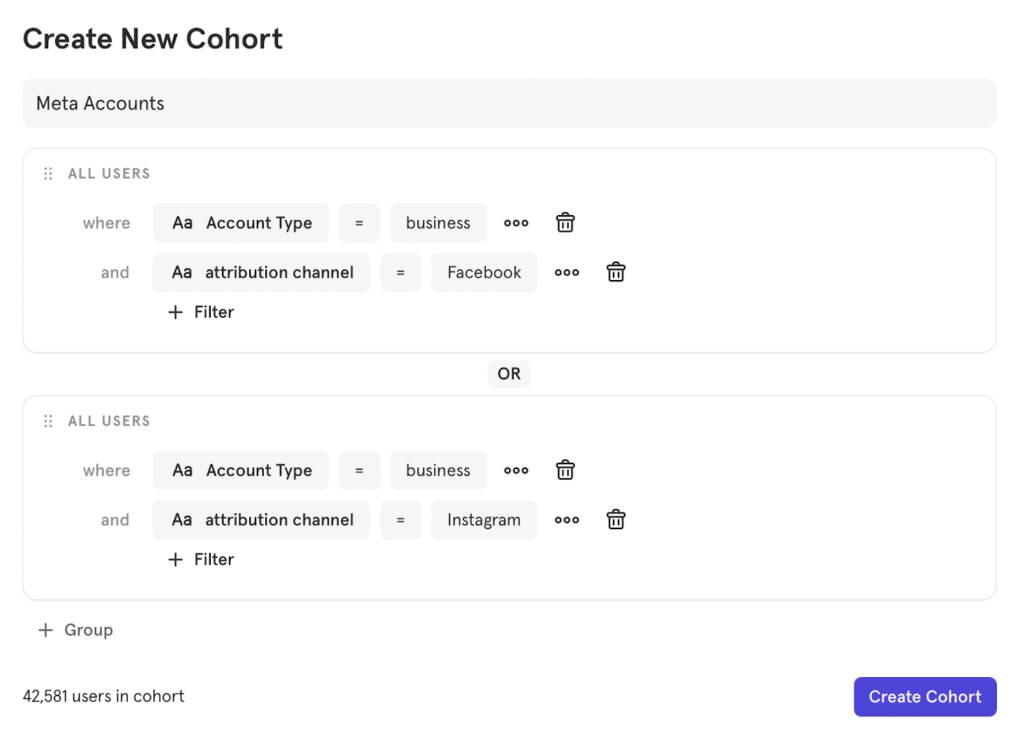
4. Analyze the results
Teams can run cohort reports as they need them, or periodically, and track the performance of cohorts against a range of factors such as:
- Retention
- Average order size
- Upgrades
- Usage
- Engagement
- Referrals
Teams can compare cohorts against one another or compare cohorts to the broader user population to understand any differences.
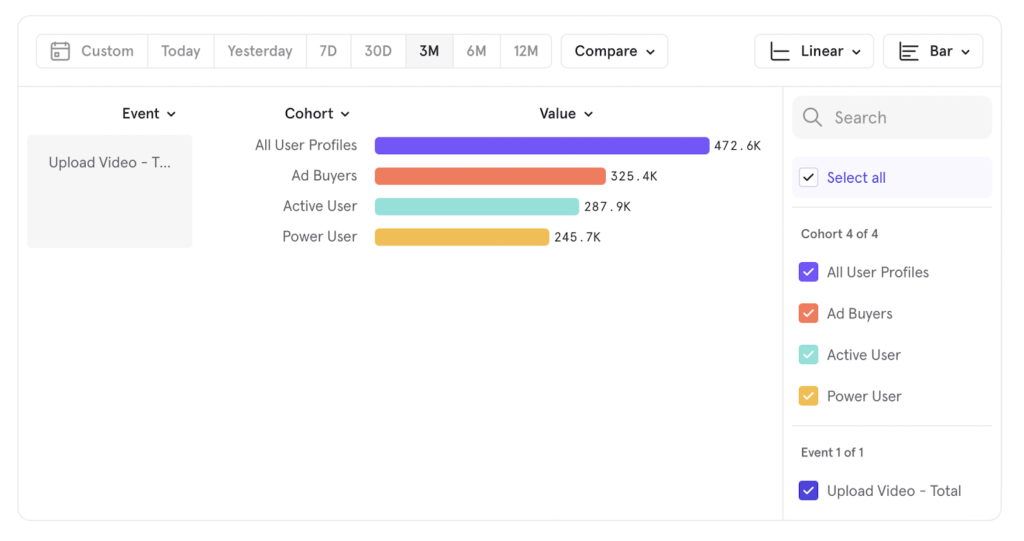
For example, cohorts that signed up for a marketing software during a particular week may exhibit far higher usage and retention than normal, and the team can investigate that cohort’s behaviors to generate ideas for improving the service.
If the team finds that those users came from a particular marketing campaign, they know to repeat that campaign. If they note that an unusual percentage of the cohort completed the onboarding sequence, the team knows to promote the onboarding sequence more aggressively.
Cohort analysis examples
Here are three real-life examples of how to use cohort analysis to improve a product:
Cohort Retention Analysis
The children’s education software CodeSpark improved its retention by dividing users into cohorts based on acquisition sources.
The team A/B tested new features with each group separately and found that new users that joined from its Hour of Code program exhibited different behaviors than those who joined during a school program.
Knowing which groups liked which features allowed the team to retain students longer.
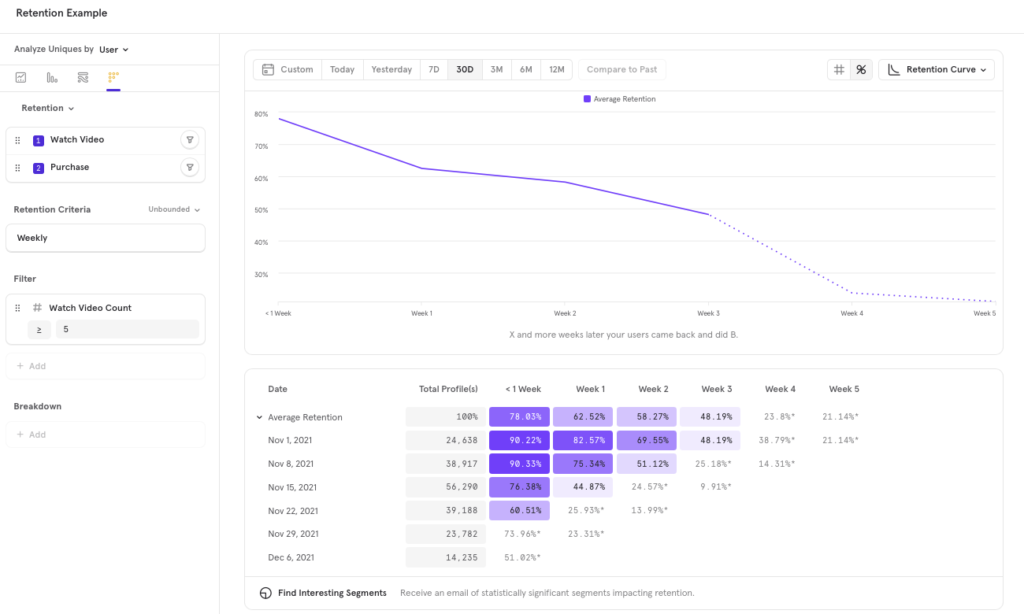
Increased revenue
The entertainment ticket sales leader Ticketmaster used Mixpanel’s user analytics to segment its B2B user base and create separate cohorts for venues, artists, and promoters.
By sending personalized messaging and A/B testing marketing campaigns to each different cohort, the marketing team significantly improved its return on marketing spend.
Improved profitability
Subscription-based media and entertainment giant STARZ-PLAY used cohorts to reduce fraud within its platform 1000x and saved 8x on its marketing spend.
The company created a cohort for users acquired through free trial promotions and found that a substantial number of users were circumventing the paywall and signing up for multiple free trials. Tracking users helped the team close the loophole and deactivate fraudulent accounts.
Ready for your own cohort analysis?
Remember: With real (and nearly real-time) data, you can make better decisions for your product, your users, your onboarding experience, and more. Interviews and feedback loops can fill in the gaps, but product analytics give the real-world actionability that no other approach can.
In short: Cohort analysis will help you improve both acquisition and retention.
Gain insights into how best to convert, engage, and retain your users with Mixpanel’s powerful product analytics. Try it free.