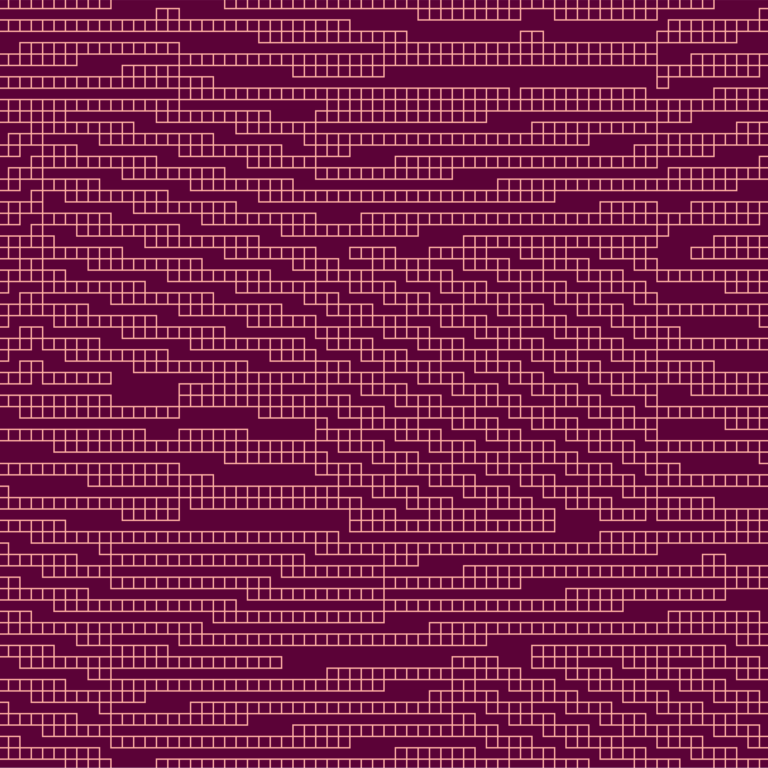
Data fatigue: Why teams stop asking questions (and how to fix it)
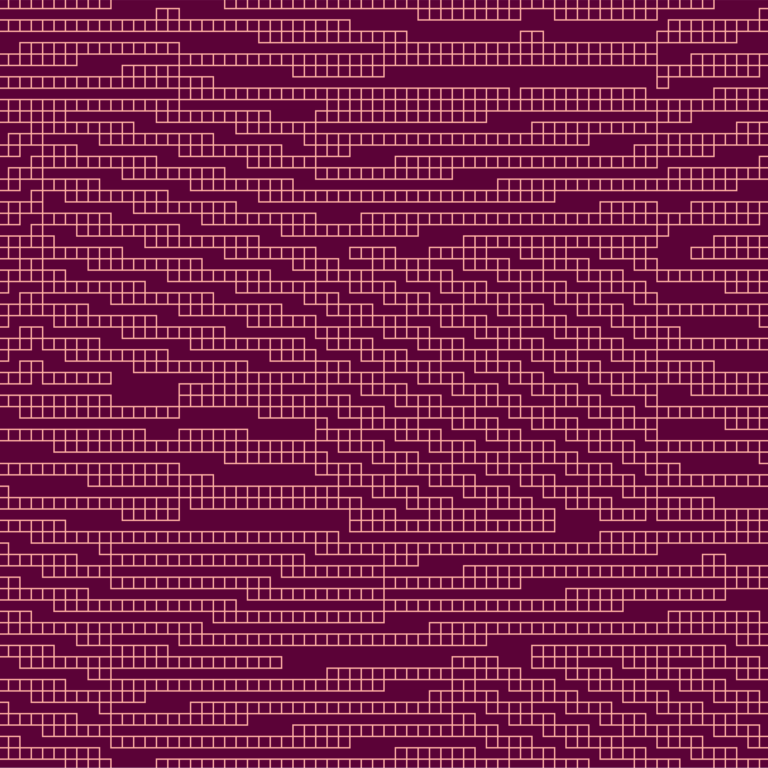
Organizations are drowning in numbers, dashboards, and reports—yet many still struggle to extract real value and insights from them.
Overwhelmed by an endless stream of metrics and no easy way to access the information they need, teams often default to surface-level analysis or abandon data-driven decision-making altogether. The result? Analysis paralysis, and a lack of certainty about the product insights that truly matter.
At Omtera, we work closely with organizations to navigate digital analytics challenges, and a recurring phenomenon I've observed is what I call "data fatigue."
What is data fatigue?
Data fatigue is a phenomenon that occurs when teams, discouraged by the complexities of data retrieval and analysis, gradually ask fewer and fewer data-related questions. Think about all your teams that need data to inform their decision-making daily. Product, CRM, Revenue—all of these teams and more need different types of data.
But most companies have a centralized data team that handles all of these data requests, and with a constant influx of requests from multiple departments, the data team struggles to keep up. This causes long wait times, backlogged requests, and frustration across the organization. As a result, teams either make decisions based on outdated insights or abandon data-driven approaches altogether in favor of gut instincts.
Over time, this leads to a culture where data-driven decision-making is stifled—not by a lack of data, but by the overwhelming processes associated with accessing and interpreting it.
How do you recognizing data fatigue? Data fatigue often manifests in subtle ways that may not impact your KPIs—yet. Some of the key signs to look for? Teams might start by asking fewer questions, relying on broad assumptions, or defaulting to generic strategies because of the perceived hassle of obtaining the specific data they actually need.
Causes of data fatigue: Why companies get stuck
Now, let’s dig a little deeper into the factors that contribute to data fatigue:
- Complex tools: Many analytics platforms require specialized knowledge to navigate, making it difficult for non-technical teams to extract insights on their own. Others, like Google Analytics, just take time to extract even basic insights.
- Company growth: As organizations scale, the focus is often on expansion rather than refining internal processes. Over time, this results in fragmented tech stacks and ad-hoc rather than strategic data workflows. Even though employees can adapt to inefficient systems, it just turns these workarounds into the norm, reinforcing inefficiencies and making it even harder to implement a structured, scalable data strategy.
- Bottlenecked data access: Dependence on a central data team is one of the most common causes of data fatigue. For example, growth teams tend to need more targeted segmentation so they can tailor UI and messaging effectively and improve the user experience. But when they depend on the data team for access to insights, they’re often forced to take the easier route—like defaulting to ad-hoc or bulk messaging instead of more custom testing.
“We used Google Analytics in the past, but it took over seven hours to prepare data for analysis, compared to seconds when using Mixpanel. We also love the ‘Alerts’ feature in Mixpanel that tells us immediately when users experience issues so we can rapidly fix them and betting can continue during high-profile sports matches.”
For example, this is something that I saw in our work with Getir (a unique company that provides convenient services like ultra-fast grocery delivery, food delivery, e-commerce, car rental, and even a ride-hailing service).
The team had been struggling with limited visibility into customer behavior and a lack of real-time insights, and we helped them implement Mixpanel to build a robust analytics ecosystem.
“With Mixpanel, we can seamlessly explore multiple scenarios and quickly answer incoming questions using its drag-and-drop capabilities. As a data team, one of its most valuable features for us is its ability to facilitate efficient A/B testing. It enables us to monitor event health post-release, quickly identify anomalies, and validate feature performance with real-time insights,” says Tuğçe Soydan, Data Analytics Manager at Getir.
3 strategies to overcome data fatigue
Imagine every team has 100 questions about the product—80 of them are relatively easy to answer. While even the best data tools may struggle with the most complex questions, teams should at least be able to get answers to that 80% quickly and effortlessly. This is where Mixpanel comes in.
In my experience, addressing data fatigue requires a multifaceted approach:
1. Invest in user-friendly tools
Adopting an intuitive analytics platform minimizes the learning curve and encourages non-technical team members to engage with your data. That way, everyone can leverage insights when they need them.
For example, eXtra, an electronics retailer in the Middle East catering to over 12 million customers, was struggling to fully leverage Google Analytics. Compounded by connectivity and data trust issues, this significantly hindered quick decision-making.
We worked with eXtra to implement Mixpanel, whose intuitive interface simplified complex queries while its server-side tracking and advanced data governance capabilities gave eXtra a reliable and actionable analytics framework.
As a result, we supported the team in achieving a 47% improvement in checkout-to-order conversion rates, and 4x increase in the exposure of analytics to non-technical teams. “Mixpanel is magical when it comes to finding answers to your questions with a well-structured data governance setup,” says Lobna Tarek, Head of Analytics at eXtra.
“I received feedback from my colleagues saying, ‘This is the best tool I’ve used in my work life.’”
2. Support internal champions
Driving a data-first culture requires leadership buy-in, and this often starts with internal champions—PMs, analytics leaders, or data-savvy team members who advocate for better tools and processes.
But to make the case, champions need to demonstrate clear ROI by showing how improved data access leads to better business outcomes. For example, a growth team with real-time user segmentation could tailor messaging more effectively, leading to higher conversion rates. Or, a product team using self-serve analytics can instantly analyze feature adoption instead of waiting days for a report, which allows them to iterate faster.
Is your data democratized? If one person is always leveraging data to make decisions—even if they’re good decisions—you might have a data accessibility issue. Why? Because, ideally, everyone should easily get the data they need.
3. Measure progress against data fatigue
One way to track improvement over time is by measuring the number of questions teams pose related to data. Teams tend to ask fewer questions when they’re not engaged, and if you’re making it easy for everyone to access the data they need, this should lead to an increase in insight-seeking.
Another important metric is a reduction in data team backlogs. As self-serve tools and streamlined processes take effect, central data teams should experience fewer routine requests because other teams can take care of (at least the basic) requests themselves.
Transform data overload into actionable insights
Data fatigue isn’t just an inconvenience—it’s a major roadblock to building a truly data-driven organization.
When teams struggle to access and interpret data, decision-making slows down, innovation stalls and gut instinct replaces real insights. But as we’ve seen, this challenge isn’t insurmountable.
By investing in user-friendly analytics tools, empowering internal champions, and tracking key indicators of progress, organizations can empower every team to ask and answer the right data questions to drive product usage, growth, and revenue.