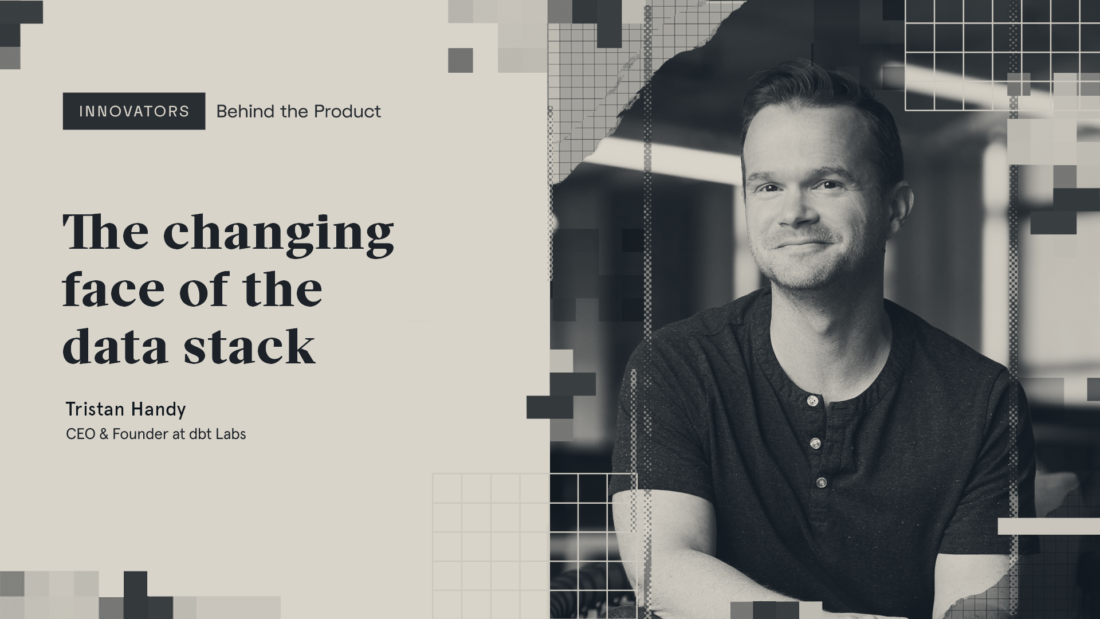
Tristan Handy on the changing face of the data stack

Tristan Handy is the founder of dbt Labs. In this interview, Tristan gives us his thoughts on the data stack of today and the hopefully improved capability and accessibility of tomorrow’s.
Ask Tristan Handy what he thinks the future of the data stack will look like and what you’ll get in return is a history lesson.
From punch cards and filing drawers to early computers. From early computer systems (basically operating on arithmetic) to the priests of Oracle and IBM. From siloed systems to open source technology that bridges the gap between data engineering and data analysis.
That’s because, as Tristan says, you’ve got to know where you’ve been in order to know where you’re going.
Having started as an “Excel guy” for hire in high school and gone on to found dbt Labs a few decades later, pioneering the practice of analytics engineering, there are few more qualified to give lessons on the past, present, and future of the modern data stack. So in our chat with Tristan, we covered all of the above, with a focus on how things like interoperability and self-serve have and will continue to define the technology.
The data-analysis gaps of yesterday and today
There have always been barriers between data and its analysis. Tristan has been on the job to see various iterations.
In his early years, when organizations weren’t collecting as much data, hand-typing figures into a spreadsheet for analysis was a bit of a labor gate. Eventually, there came technologies to collect things like software user events automatically, which made data far more plentiful and turned the process of rendering sense of what you’ve gathered into the obstacle. That birthed a whole new suite of job titles and tools.
Tristan breaks it down: “There were data analysts and data engineers. And there was a wall between the two of them. Engineers are empowered to build data pipelines while analysts actually knew what the heck the business did and the questions they were trying to answer.”
It was a silo on steroids: Analysts would need new data or tweak the data they’re looking at in order to answer a specific question. Instead of being able to dig into the data themselves, they had to ship a request over to data engineers. “And then they would say, ‘I’ll get to it in two days or two weeks or two months,'” Tristan explains.
The result has been less than surprising. “There was a tremendous amount of queue-based tension between these two teams.” It’s why Tristan built dbt in the first place: to break down the wall by allowing data analysts, with their technical skills, to self-serve on the creation and analysis of datasets.
Read: Shreyas Doshi on choosing, refining, and tracking product metrics
For Tristan, it’s about the recognition that “the analytical process is also fundamentally an engineering process. Not only do you have to answer that question once, but you have to push your analysis into production so that it is constantly going to be live from then on out.”
Today, Tristan says product analytics tools like Mixpanel can return deep analysis of specific user and product topics through simplified, self-serve data questions—an operation that is “way worse in practice” through data visualization business intelligence (BI) tools, like Looker or Mode, he explains. But, when it comes to breadth of analysis, it can still be necessary to implement BI tools in your stack, which leaves a lot of room for friction in many types of standard data analysis.
Tristan gives this example: “If you want to create a good retention rate cohort analysis, it takes you about 80 lines of cleanly formatted SQL, and there’s probably four or five common table expressions in there.”
He’s trained a lot of people to essentially write that query. “It’s one of the hardest things that I’ve had to train people on,” he says. “You write it, use it for a day, and then you don’t have to use it for three months. By that time, you totally forget how to write the query again.”
Part of the complexity is writing the query in the first place, but it’s mostly all the iterations of the query you need for subsequent analysis. “Maybe you want to look at the retention rate a little bit differently based on a different event. And so you find yourself trying to add all this interactivity. You’re essentially building a product, but the product has to conform to the interface of your BI tool.”
Not only that, but it also runs on a 10+ second delay because you’re going back and forth with your data warehouse.
All that to say: Though some tools and platforms are doing a good job of bridging some of that gap between the proverbial data engineer and data analyst, there’s still progress ahead to be made at large.
“If you want to create a good retention rate cohort analysis [with BI tools], it takes you about 80 lines of cleanly formatted SQL, and there’s probably four or five common table expressions in there … You’re essentially building a product.”
Interoperability is the key to moving the data stack and products forward
One way Tristan believes we can make that progress: Tools need to move even more toward open source and interoperability, which will push data stack maturity forward.
He’s written about how the standardization around SQL did some of that last decade. Having a common data language meant that products of all data functions could work better together. And even for all the woes SQL might bring any non-engineer data practitioners today, that same kind of standardization model around a—let’s hope friendlier—protocol in the future could open up much more access.
In the meantime, the trend of centralizing data in a cloud-based warehouse has opened new opportunities for companies to “à la carte” their stack from all of today’s SQL-loving tools, says Tristan. “That eliminates a lot of the data lock-in where the data is stuck in this tool or in that tool. It’s easier to switch if you’re adopting the modern data stack.”
The shift to interoperability, and companies getting to create the best solutions for their needs, has taken a couple of decades: “You were in a duopoly inside of enterprise software: Microsoft and Oracle. And you were either a Java shop with Oracle technologies, or you were a .net shop with Microsoft technologies. But then, over the next 10 years, things open up.”
Linux, says Tristan, is what made the difference for software engineers. It became the “foundation that everything else gets built on top of.”
With Linux as the base, other open source projects like Docker and Kubernetes paved the way forward. “The primary stance that all software companies now need to take is one of openness.”
Building a more accessible front-end to match a more sophisticated back-end
Of course, as we stack more and more data-processing products together, functionality increases, but so does the complexity involved with a user (product manager, engineer, marketer) interfacing with them all together.
This is no new problem when it comes to human-computer interaction. Tristan points out that it wasn’t until the iPhone came along that many people got their first dose of access to cloud-stored information and high computational power.
“I don’t think we should be super surprised that an increase in capability with the modern cloud data platform is paired with an increase in inaccessibility of that capability,” Tristan says. “It just means that we, as an industry, need to invent tools to make this new paradigm accessible to people who would find it useful.”
Tristan has high hopes for the future of the data stack having its own iPhone moment. And as for what it might be, he’d love to see solutions for replacing the last vestiges of the spreadsheet way of doing data.
“The advancement that we saw in computer interfaces in the latter half of the 20th century was an increase in technological sophistication, but a decrease in end-user complexity.”
“If you look at business intelligence tools, these are products that think about the world in terms of tables, rows, and columns,” Tristan bemoans.
It’s not how we think naturally, in other words. Just like how using a mouse to point at something on a computer screen is less natural than physically pointing to it with your finger on an iPhone.
“Imagine being able to actually apply an understanding of semantic understanding of the business domain to the analysis that you’re doing,” Tristan explains. “You can say things like, ‘Here are things that happened in the product, and here’s a useful view of it because I know the kinds of things I want to learn from my data.'”
That approach makes analytical work more accessible, which spells the future of the data stack—and not just for product analytics
“Product analytics is a great use case for this since it’s both high-value and one of the more complex data domains. But I think that there are other very good use cases and vertical analytics solutions.” Accounting data, for example, could benefit from a more semantic approach to analytics.
With that in mind, Tristan thinks self-serve will see the biggest flux during the maturation of the data stack. Even if accessibility faces some challenges as data gets more complex, the interface will catch up.
“I don’t think it’s that [self-serve analytics] are going to get more ‘complex’—it’s that they’re going to get more ‘sophisticated,'” Tristan tells us. He goes back to personal computing for another example: “The advancement that we saw in computer interfaces in the latter half of the 20th century was an increase in technological sophistication, but a decrease in end-user complexity.”
Put another way: The windowed interface was significantly harder to pull off technically, but far simpler for users. This is the kind of maturation that the modern data stack still needs to go through.
“The industry is building all of the necessary underpinning technology right now to enable that to happen: scalable data processing and storage and data transport pipes,” says Tristan. “The user experience innovations that take advantage of all of these new technologies will give way to building the more user-friendly, but also more technically sophisticated, front-end.”
In the meantime, pieces are coming together to make the data stack accessible (like Mixpanel and dbt, for example). The industry is still working to create the best front-end solutions for a unified data stack.
Tristan’s own analysis: He’s excited to see exactly what’s to come.
“It seems inevitable that as UX improves, more professionals in all fields will make use of data. I think it’s impossible, and not productive, to attempt to imagine what all of these potential uses could be.”
About Tristan Handy
Tristan has been a data practitioner for over two decades. Currently the founding CEO at data transformation solutions company dbt Labs, he’s also held leadership positions at RJMetrics and Squarespace.